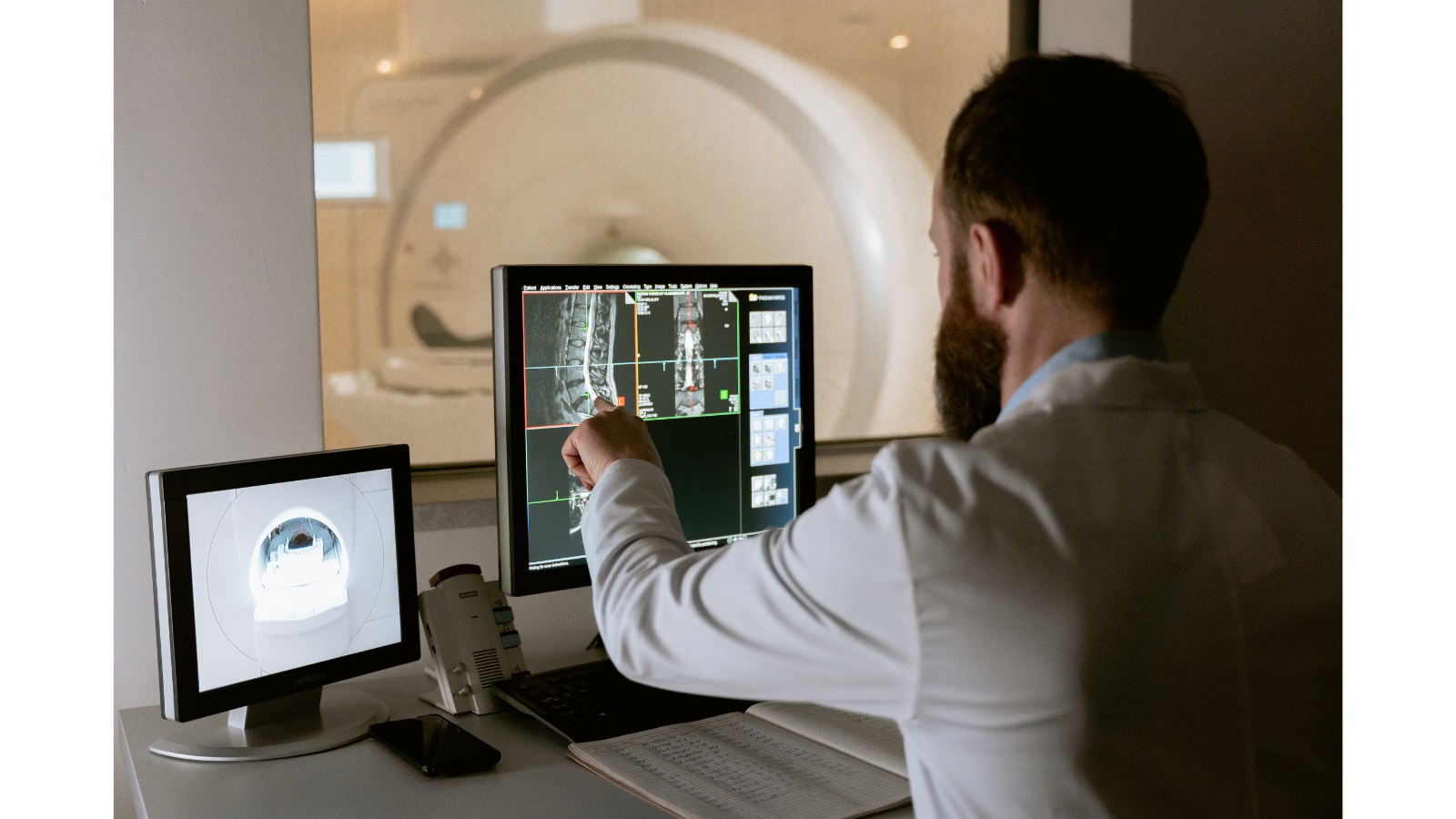
Researchers from Johns Hopkins and Columbia University presented a groundbreaking technique at the Neural Information Processing Systems Conference to enhance AI and ML models’ performance in medical note analysis. Addressing the challenge of dataset shift in healthcare AI, the team developed a data augmentation approach using large language models. By making models less sensitive to variations in clinicians’ writing styles, the technique significantly improves robustness. Leveraging counterfactual data generated by LLMs, the researchers demonstrated the method’s effectiveness in improving generalizability across diverse healthcare settings. This innovation contributes to the broader goal of establishing an AI safety framework for healthcare applications.
The deployment of large language models (LLMs) in healthcare settings offers a promising solution to the challenges posed by the extensive and intricate clinical notes found in electronic health records (EHRs). A team of researchers from Johns Hopkins University and Columbia University has presented a groundbreaking technique at the 37th Annual Conference on Neural Information Processing Systems. This technique focuses on improving the performance, generalizability, and safety of artificial intelligence (AI) and machine learning (ML) models utilized for medical note analysis, tackling the prevalent issue of dataset shift in healthcare AI.
Dataset Shift in Medical Note Analysis:
Clinical notes within EHRs contain valuable data that, if properly analyzed, could significantly enhance patient care. However, the complexity and sheer volume of this information make manual review and analysis impractical. AI technologies hold the potential to swiftly process vast amounts of data, but issues related to model generalizability and performance hinder their seamless deployment.
AI and ML models for medical note analysis are typically trained on specific health systems’ EHR data. The challenge arises from the inherent variability in medical notes across different health systems, leading to what is known as a “dataset shift.” This phenomenon raises concerns about the safety and efficacy of deploying healthcare AI models.
The Role of Writing Styles and Templates:
The research team emphasizes that variations in clinicians’ writing styles can result in AI models incorrectly inferring associations between factors like grammar or word choice and a patient’s diagnosis. Similarly, the use of templates, including tables and headings, can introduce biases into the models. Clinicians may use these templates frequently, potentially leading AI models to learn from spurious correlations rather than genuine associations in the data.
Data Augmentation for Model Generalizability:
To address these challenges, the researchers propose a novel data augmentation technique designed to enhance the generalizability of AI models. The key insight is that making models less sensitive to changes in writing habits and styles between different caregivers can significantly improve their robustness.
The team achieves this by leveraging large language models (LLMs) to generate counterfactual data. Instead of having clinicians manually rewrite each other’s notes, the researchers employ LLMs to answer the question, “How would this note look had Dr. Beth written it?” This approach generates diverse writing styles for the same medical note, allowing the AI model to focus on the content rather than the style or templates used.
Using auxiliary data from clinical notes, such as patient demographics, timestamps, and document types, helps generate high-quality approximations of counterfactual data. This data augmentation technique demonstrates a healthcare domain-informed approach to using LLMs and significantly improves the generalizability of AI models for medical note analysis.
Application in Real-World Settings:
The utilization of causally motivated data augmentation methods like the one proposed by the researchers holds great potential for addressing challenges in developing robust and reliable ML systems, especially in safety-critical applications. The team’s efforts contribute to the larger goal of establishing an AI safety framework for healthcare applications.
Medical Note Analysis and AI in Clinical Documentation:
The incorporation of AI in medical note analysis is crucial for advancing the clinical documentation improvement process. EHR documentation assistants, often based on scribe- or voice-based technologies, are increasingly employed by health systems to streamline documentation and alleviate clinician burnout. These tools aim to reduce the time clinicians spend on documentation without compromising the quality of the notes.
Medical note analysis goes beyond documentation efficiency; it serves as a gateway to extracting valuable associations within the clinical data. By surfacing potential relationships, AI models can inform predictive analytics and guide clinical decision-making. The collaborative efforts of interdisciplinary teams in the development of advanced AI techniques contribute to the ongoing evolution of healthcare AI applications.
The presented data augmentation technique represents a significant advancement in addressing challenges related to model generalizability in AI-driven medical note analysis. By leveraging large language models and counterfactual data generation, the researchers have demonstrated a practical approach to mitigate the impact of dataset shifts and improve the robustness of AI models.
As healthcare systems continue to embrace AI technologies for clinical documentation improvement, the importance of ensuring the generalizability and safety of these models cannot be overstated. The causally motivated data augmentation methods introduced in this research offer a promising avenue for enhancing the reliability of machine learning systems in safety-critical healthcare applications.
The ongoing collaboration between researchers, clinicians, and AI experts is crucial for developing comprehensive AI safety frameworks that can be applied across various text classification tasks in healthcare. As the field progresses, the integration of innovative AI techniques will play a pivotal role in shaping the future of medical note analysis and its broader implications for healthcare AI.