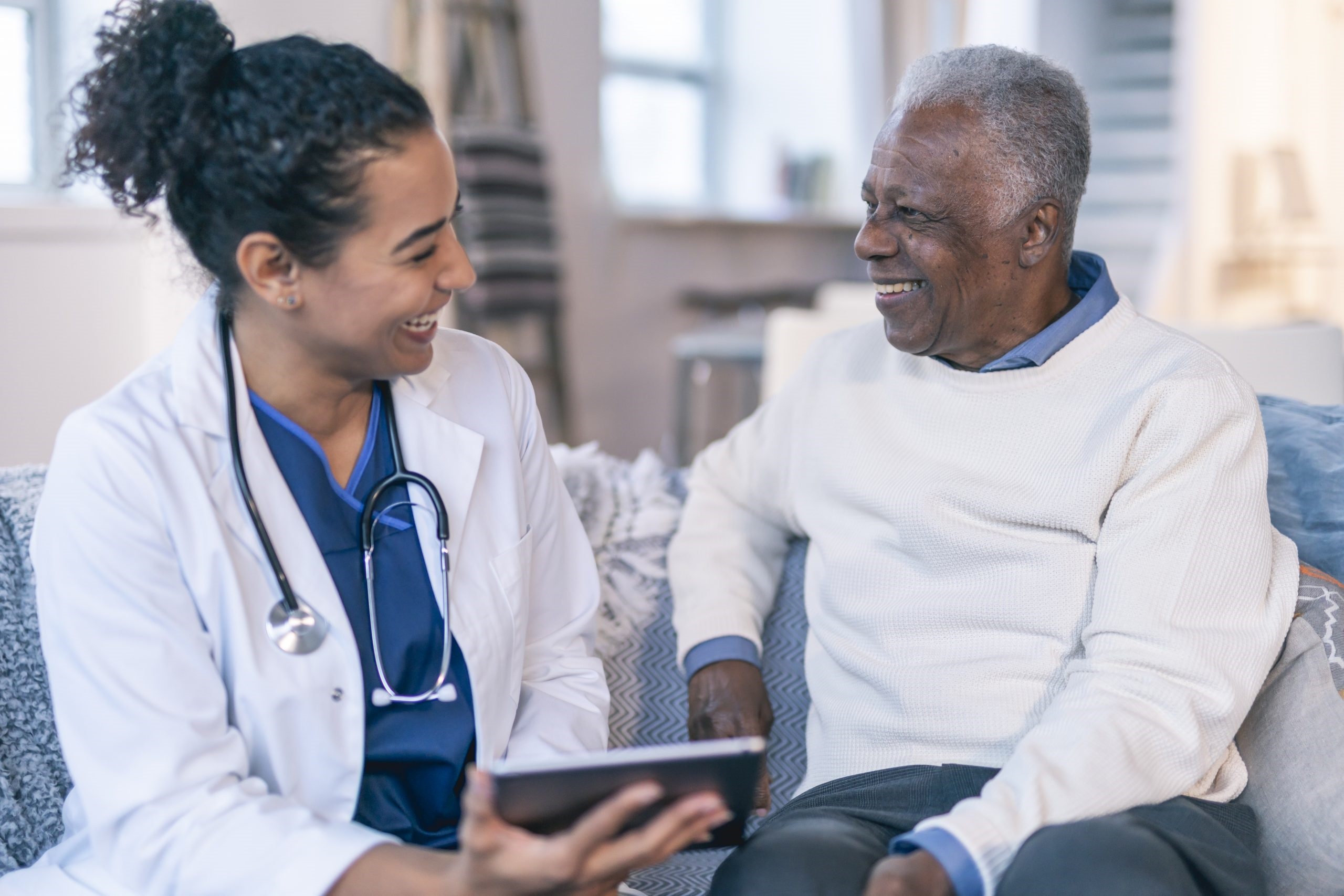
Scholars from UCSD argue for a shift towards prioritizing patient outcomes in healthcare AI regulation. The current focus on process-centric regulations overlooks the fundamental goal of improving patient health. They propose an outcomes-centric approach, advocating for rigorous evaluations grounded in clinical evidence. Despite potential challenges, such as regulatory complexities, establishing a dedicated federal agency could facilitate the implementation of outcome-centric regulations. By embracing this approach, policymakers can ensure the safe and effective deployment of AI technologies in healthcare, ultimately enhancing patient care and outcomes.
In the realm of healthcare AI regulation, the emphasis on process-centric regulations has overshadowed the crucial aspect of patient outcomes. Despite efforts to ensure safety, quality, and equity, the absence of a robust evaluation framework leaves room for suboptimal performance and potential harm to patients. Scholars from UCSD contend that the ultimate goal of medicine is to save lives, necessitating a paradigm shift towards prioritizing patient outcomes in AI regulation. This viewpoint underscores the imperative of aligning regulatory efforts with the core objective of improving patient health through innovative technologies.
In a recent viewpoint published in the Journal of the American Medical Association (JAMA), scholars from the University of California San Diego (UCSD) highlighted the necessity for healthcare AI regulations to prioritize patient outcomes. They critiqued the White House Executive Order on the Safe, Secure, and Trustworthy Development and Use of Artificial Intelligence (AI), arguing that while it addresses safety, quality, privacy, and equity, it overlooks the crucial aspect of patient outcomes.
Lead author Davey Smith, MD, emphasized that the ultimate aim of medicine is to save lives, underscoring the importance of AI tools demonstrating tangible improvements in patient outcomes before widespread adoption. However, the current executive order primarily focuses on what the authors termed “process-centric” regulations, which dictate compliance procedures for developers rather than assessing the actual impact on patient health.
The distinction drawn by the researchers between “outcomes-centric” and “process-centric” regulations is pivotal. While process-centric regulations are common in various industries, especially for ensuring quality control, the unique nature of healthcare AI necessitates an outcomes-centric approach. Unlike traditional products, AI technologies in healthcare evolve rapidly, making static process-centric regulations inadequate and potentially obsolete.
Moreover, the authors pointed out that the executive order fails to consider existing regulatory pathways, such as those established by the Food and Drug Administration (FDA), which already prioritize patient outcome assessments for drugs and medical devices. This oversight becomes particularly evident when examining case studies such as early warning systems for sepsis. Despite their widespread deployment, these systems may fall short in clinical efficacy, highlighting the need for outcome-centric evaluations before market introduction.
The authors suggested revising the executive order to prioritize patient outcomes in AI product regulation, akin to the rigorous standards applied to pharmaceuticals. They proposed that AI models should undergo evaluations grounded in clinical evidence, including randomized clinical trials, to ensure meaningful improvements in patient outcomes compared to existing standards.
While acknowledging potential barriers to implementing an outcome-centric approach, such as resource constraints and regulatory complexities, the researchers proposed the establishment of a dedicated federal agency to facilitate clinical AI evaluation. Such an agency could develop rules, standards, and approval mechanisms specific to digital health technologies, mitigating regulatory challenges and fostering innovation.
Despite the complexities involved, the authors remained optimistic about the feasibility of regulating healthcare AI to prioritize patient outcomes without stifling innovation. They emphasized the digital nature of AI models, which enables faster assessment and iterative improvements compared to traditional drug trials.
In essence, the argument put forth by UCSD scholars highlights the necessity of reorienting healthcare AI regulation to focus on patient outcomes. By shifting from process-centric to outcomes-centric approaches, policymakers can better address the fundamental purpose of healthcare – saving lives and improving patient well-being. While challenges may arise in implementation, such as regulatory complexities and resource constraints, the establishment of a dedicated federal agency could facilitate the adoption of outcome-centric regulations. Ultimately, embracing this approach will not only ensure the safe and effective deployment of AI technologies but also drive tangible improvements in patient care and outcomes.