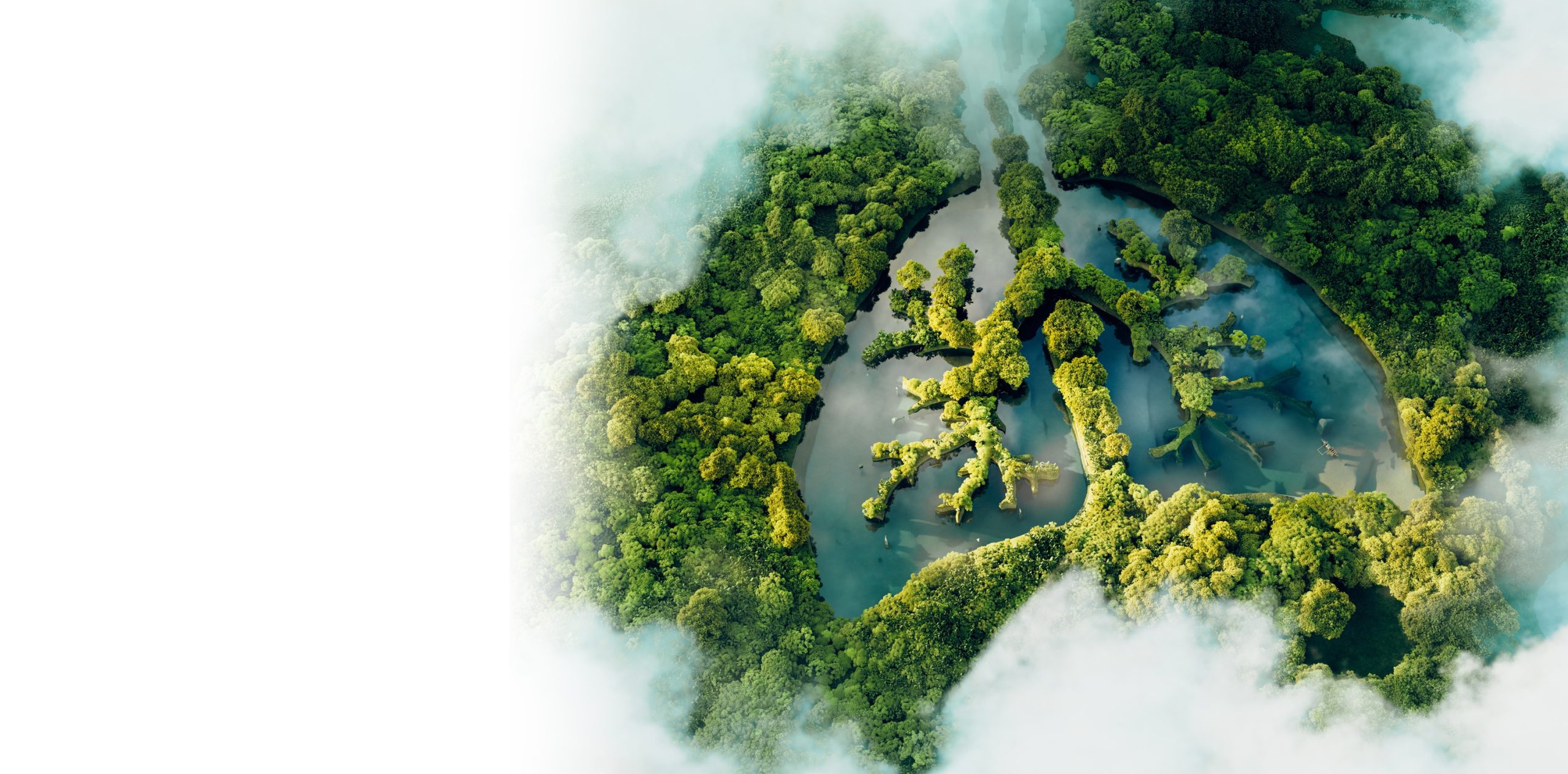
The integration of artificial intelligence (AI) in radiology presents a paradigm shift, offering both transformative benefits and environmental challenges. This discourse explores the multifaceted impacts of AI on the ecological landscape, emphasizing the imperative to reconcile technological advancements with environmental stewardship. Strategies encompassing computational efficiency, energy-conscious hardware selection, and collaborative partnerships are advocated to mitigate the environmental footprint of AI. Through a concerted effort to foster sustainability, stakeholders can navigate the complexities of AI integration in radiology while advancing ecological integrity.
In the contemporary landscape of radiology, the integration of artificial intelligence (AI) brings forth a pivotal juncture where technological advancements intersect with environmental responsibility. Highlighting this intersection, a recent focus article in Radiology, a journal of the Radiological Society of North America (RSNA), underscores the imperative to contemplate the environmental ramifications of AI tools. As healthcare and medical imaging activities contribute significantly to greenhouse gas (GHG) emissions, researchers emphasize the necessity to assess and address the ecological footprint accompanying the proliferation of AI in radiological practices.
Dr. Kate Hanneman, alongside a team of researchers, sheds light on the multifaceted impacts of AI integration in radiology. While AI promises enhanced workflows, expedited image acquisition, cost reduction, and improved patient experiences, its adoption comes with a notable downside—the substantial energy consumption entailed in the development and deployment of AI models and the storage of associated data. The collective endeavor to harness AI’s benefits while mitigating its adverse environmental effects emerges as a central theme in this discourse.
The process of developing AI models demands copious amounts of training data, necessitating extensive data storage infrastructure within healthcare institutions. With many institutions resorting to cloud storage solutions, the environmental toll of housing data in energy-intensive data centers is exacerbated. The magnitude of this challenge becomes apparent as recent estimates position global GHG emissions from data centers surpassing those of the airline industry. Yet, amidst this sobering reality lies a glimmer of hope—a call to action to explore avenues for sustainability and efficiency.
Efforts to curtail the environmental impact of AI in radiology encompass a spectrum of strategies, ranging from technological interventions to collaborative initiatives. By optimizing AI algorithms for computational efficiency, selecting energy-efficient hardware, employing data compression techniques, and implementing tiered storage systems, healthcare providers can mitigate energy consumption and minimize GHG emissions. Moreover, strategic partnerships with providers leveraging renewable energy sources offer a pathway toward fostering sustainability in AI infrastructure.
The geographical location of data centers emerges as a pivotal determinant of their environmental sustainability, with cooler climates and proximity to renewable energy sources offering inherent advantages. Embracing a collaborative ethos, healthcare institutions are encouraged to pool resources and leverage collective expertise to distribute the environmental burden of data storage infrastructure more equitably. Such collaborative efforts not only amplify the efficacy of sustainability initiatives but also foster a culture of shared responsibility in environmental stewardship.
Despite the laudable strides towards sustainability, challenges and knowledge gaps persist on the journey to reconcile AI advancements with environmental imperatives. Limited data on radiology-specific GHG emissions, resource constraints, and regulatory complexities underscore the multifaceted nature of this endeavor. However, amidst these challenges lies an opportunity to redefine metrics of success in healthcare and medical imaging, wherein environmental sustainability assumes parity with financial considerations.
Dr. Hanneman articulates a vision wherein sustainability becomes an intrinsic quality metric in the decision-making framework surrounding AI and radiology. By cultivating mindfulness and awareness regarding energy usage and GHG emissions, stakeholders can navigate the complexities of AI integration while advancing environmental stewardship. Ultimately, the convergence of technological innovation and environmental responsibility beckons stakeholders in radiology to embark on a collective journey towards a sustainable future—one where the promise of AI is realized without compromising ecological integrity.
Overall, In the quest for technological innovation, environmental sustainability must not be relegated to the periphery. The discourse surrounding the environmental impacts of artificial intelligence (AI) in radiology underscores the imperative for stakeholders to engage in a delicate balancing act—one that optimizes the transformative potential of AI while safeguarding the planet for future generations. Through strategic interventions, collaborative partnerships, and a steadfast commitment to sustainability, the radiology community can chart a course toward a future where healthcare innovation and environmental stewardship converge harmoniously.