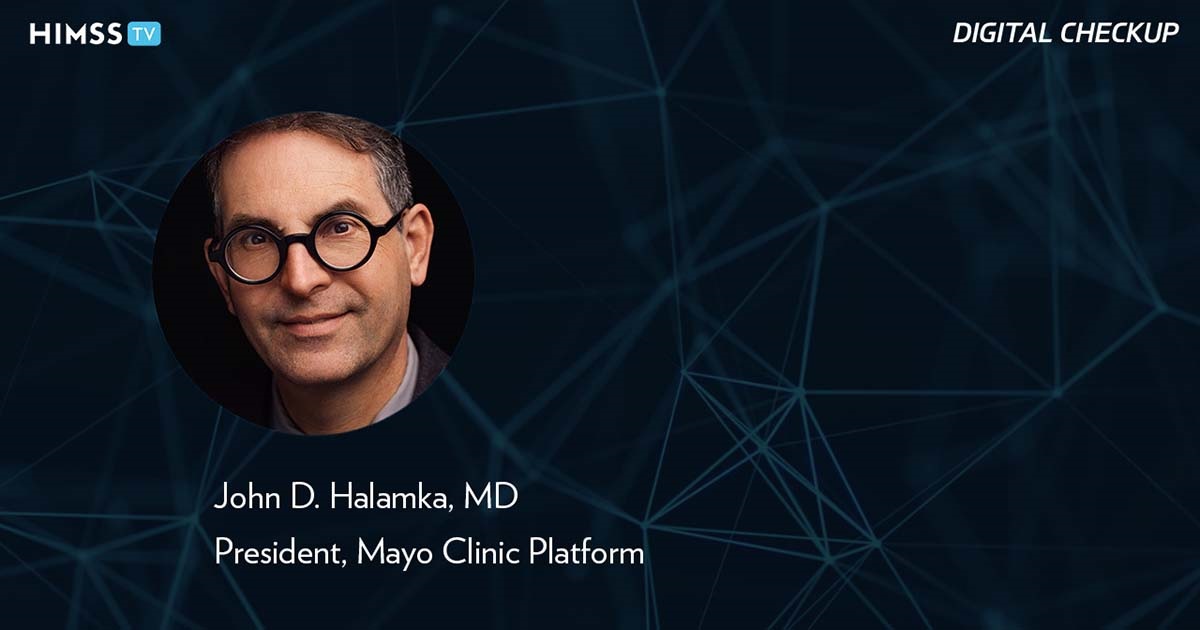
Dr. John Halamka’s discourse at HIMSS24 provided a comprehensive examination of the opportunities and challenges surrounding predictive and generative artificial intelligence (AI) in clinical settings. Addressing issues of transparency, data quality, ethical considerations, and global collaboration, Halamka outlined a roadmap for stakeholders to navigate the complexities of healthcare AI effectively. By emphasizing the importance of rigorous evaluation frameworks, collaborative initiatives, and ethical standards, Halamka underscored the imperative of ensuring patient safety while harnessing the transformative potential of AI in healthcare.
In his keynote address at HIMSS24, Dr. John Halamka, president of the Mayo Clinic Platform, delivered an incisive analysis of the risks and benefits associated with predictive and generative AI in clinical practice. Acknowledging the immense potential of these technologies to revolutionize healthcare delivery, Halamka also highlighted the critical need for transparency, accountability, and ethical considerations in AI development and deployment. Grounded in his extensive experience at the Mayo Clinic and collaborative efforts with the Coalition for Health AI, Halamka’s insights provided invaluable guidance for stakeholders navigating the intricate landscape of healthcare AI.
Addressing the credibility challenges faced by healthcare AI, Halamka raised poignant questions about the opacity of many AI models. He urged stakeholders to scrutinize the training data, consistency, reliability, and clinical trial validation of predictive algorithms currently in use. Recognizing the imperative of ensuring a safe AI future, he underscored the importance of adopting strategies to mitigate risks effectively.
Central to the discussion is the foundational role of high-quality data. Halamka stressed the necessity of curated, normalized data acquisition, acknowledging the complexity of data integrity within electronic health record systems. He cautioned against the potential biases inherent in poorly gathered social determinants of health data, underscoring the importance of comprehensive and representative datasets.
Moreover, Halamka urged stakeholders to consider the diversity and depth of data elements, emphasizing the significance of multimodal data sources beyond structured patient registration. Evaluating data breadth, encompassing a sufficiently large patient cohort is crucial for developing resilient AI models capable of delivering meaningful insights across diverse populations.
Highlighting the global nature of healthcare challenges, Halamka emphasized the inadequacy of institution-specific datasets for creating universally applicable algorithms. He advocated for collaborative, federated approaches involving diverse participants to foster model creation, testing, and refinement on a global scale.
However, significant hurdles remain, particularly in regions with limited digitization of healthcare records. Halamka cited the example of Switzerland, renowned for its chocolate but hindered by paper-based health records, underscoring the pressing need for digital transformation to enable widespread AI implementation.
Ethical considerations loom large in AI model development, particularly concerning the inclusion of sensitive variables such as race and ethnicity. Halamka cautioned against inadvertently perpetuating biases and emphasized the importance of rigorous testing and evaluation frameworks to ensure fairness and effectiveness.
Drawing on the Mayo Clinic’s experience, Halamka underscored the necessity of robust assurance processes to identify and mitigate algorithmic biases. He cited the example of a cardiology algorithm exhibiting performance disparities based on body mass index, illustrating the ethical complexities inherent in AI deployment.
In response to these challenges, Halamka highlighted the collaborative efforts of the Coalition for Health AI in defining standards for fairness, effectiveness, and safety in AI. He emphasized the value of shared metrics and artifacts to guide stakeholders in navigating the complexities of AI deployment.
Looking ahead, Halamka stressed the evolving landscape of generative AI evaluation, noting the unique challenges posed by these technologies. With an emphasis on scrutiny and rigorous validation, he urged stakeholders to tread cautiously in harnessing the potential of AI while prioritizing patient safety.
Dr. John Halamka’s elucidation of the complexities inherent in healthcare AI served as a clarion call for stakeholders to adopt a principled approach toward technology integration. By prioritizing transparency, data quality, and ethical considerations, stakeholders can mitigate risks and maximize the benefits of AI in healthcare delivery. Through collaborative initiatives such as the Coalition for Health AI, Halamka underscored the importance of collective action in defining standards and best practices for AI development and deployment. Ultimately, Halamka’s insights offer a roadmap for stakeholders to navigate the evolving landscape of healthcare AI while upholding the paramount principle of patient safety.