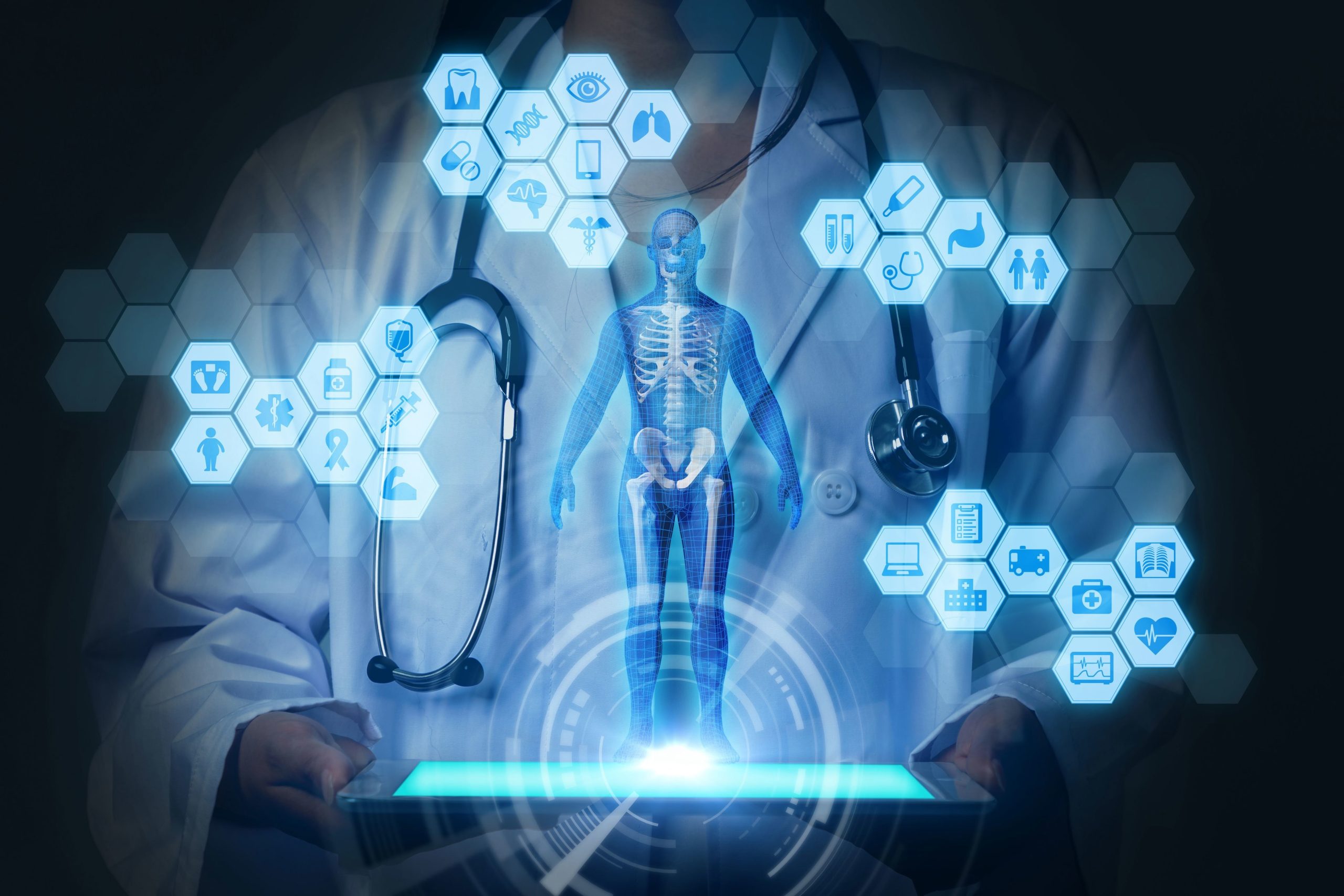
Innovations in machine learning are reshaping precision medicine, offering rapid predictions of immune responses and patient outcomes. The introduction of tools like HLA-Inception enables clinicians to tailor treatments based on individual molecular interactions, improving efficacy and reducing adverse effects. By analyzing thousands of MHC-1 alleles and leveraging electrostatic signatures, researchers pave the way for personalized treatment plans, particularly in cancer therapy. The integration of machine learning accelerates the accessibility of novel treatments, democratizing healthcare and enhancing patient care across diverse medical conditions. As machine learning continues to evolve, its role in precision medicine promises to revolutionize healthcare delivery and improve patient outcomes globally.
Machine learning plays a pivotal role in advancing precision medicine, particularly in predicting how individual immune systems respond to foreign cells. Recent research introduces HLA-Inception, a novel machine-learning model designed to analyze molecular interactions and forecast immune responses. The complexity of MHC-1 interactions poses significant challenges, but insights gleaned from HLA-Inception offer a promising avenue for personalized treatment plans, especially in cancer therapy. By accelerating the prediction of immune responses, machine learning enhances accessibility to tailored treatments, transforming healthcare delivery and improving patient outcomes worldwide.
Machine learning (ML) has emerged as a powerful tool in advancing precision medicine, particularly in predicting how individual immune systems respond to foreign cells. Recent research from Arizona State University introduces a novel ML model, HLA-Inception, designed to analyze molecular interactions and predict immune responses.
Understanding Major Histocompatibility Complex-1 (MHC-1) Proteins
MHC-1 proteins are integral to the immune system’s ability to recognize and respond to foreign entities. These proteins, present on cell surfaces, bind and display foreign peptides, facilitating immune recognition and subsequent attack.
Challenges in Predicting Immune Response
The diversity of MHC-1 genes and alleles poses a significant challenge in developing accurate prediction models. With thousands of MHC-1 variants in the human population, each interacting with peptides differently, creating a predictive model becomes complex.
Development of HLA-Inception
To address the complexity of MHC-1 interactions, researchers analyzed thousands of MHC-1 alleles, identifying patterns in peptide binding preferences. Leveraging electrostatic signatures on protein surfaces, they categorized MHC-1 into 11 distinct classes, facilitating the prediction of immune responses.
Impact on Immunotherapy and Precision Medicine
Insights from HLA-Inception extend beyond predicting immune responses to offering insights into patient outcomes, particularly in cancer treatments. Individuals with a diverse range of MHC-1 variants exhibit higher survival rates with certain cancer therapies, highlighting the potential of personalized treatment plans.
Accelerating Precision Medicine
The development of HLA-Inception represents a significant advancement in precision medicine, offering rapid predictions of immune responses and patient outcomes. Lead author Abhishek Singharoy emphasizes the transformative impact of this tool, reducing prediction times from days to seconds.
Personalized Treatment Plans
The integration of HLA-Inception into clinical practice holds promise for personalized treatment plans, particularly in conditions like cancer. By accurately predicting immune responses, clinicians can tailor treatments to individual patients, improving efficacy and reducing adverse effects.
Enhancing Accessibility of Treatments
Eric Wilson highlights the broader implications of ML in healthcare, emphasizing its role in de-risking and personalizing treatments. ML and AI-based tools democratize access to novel therapies, eliminating the need for costly experiments to determine treatment suitability.
Expanding Applications of ML in Precision Medicine
The application of ML-based tools in precision medicine continues to expand, with researchers exploring diverse medical domains.
Predicting Brain Metastasis in Lung Cancer
Researchers from Washington University School of Medicine employ deep learning (DL) to predict brain metastasis risk in non-small cell lung cancer (NSCLC) patients. By analyzing histopathology images, DL algorithms identify abnormal features, outperforming traditional methods in flagging high-risk patients.
Machine learning’s integration into precision medicine represents a paradigm shift, offering rapid predictions of immune responses and patient outcomes. Tools like HLA-Inception empower clinicians to personalize treatment plans based on individual molecular interactions, enhancing efficacy and reducing adverse effects. By analyzing diverse MHC-1 alleles and leveraging electrostatic signatures, machine learning accelerates the accessibility of tailored treatments, revolutionizing healthcare delivery. As machine learning continues to evolve, its role in precision medicine promises to reshape healthcare practices, driving improved patient outcomes and transforming medical treatment paradigms globally.