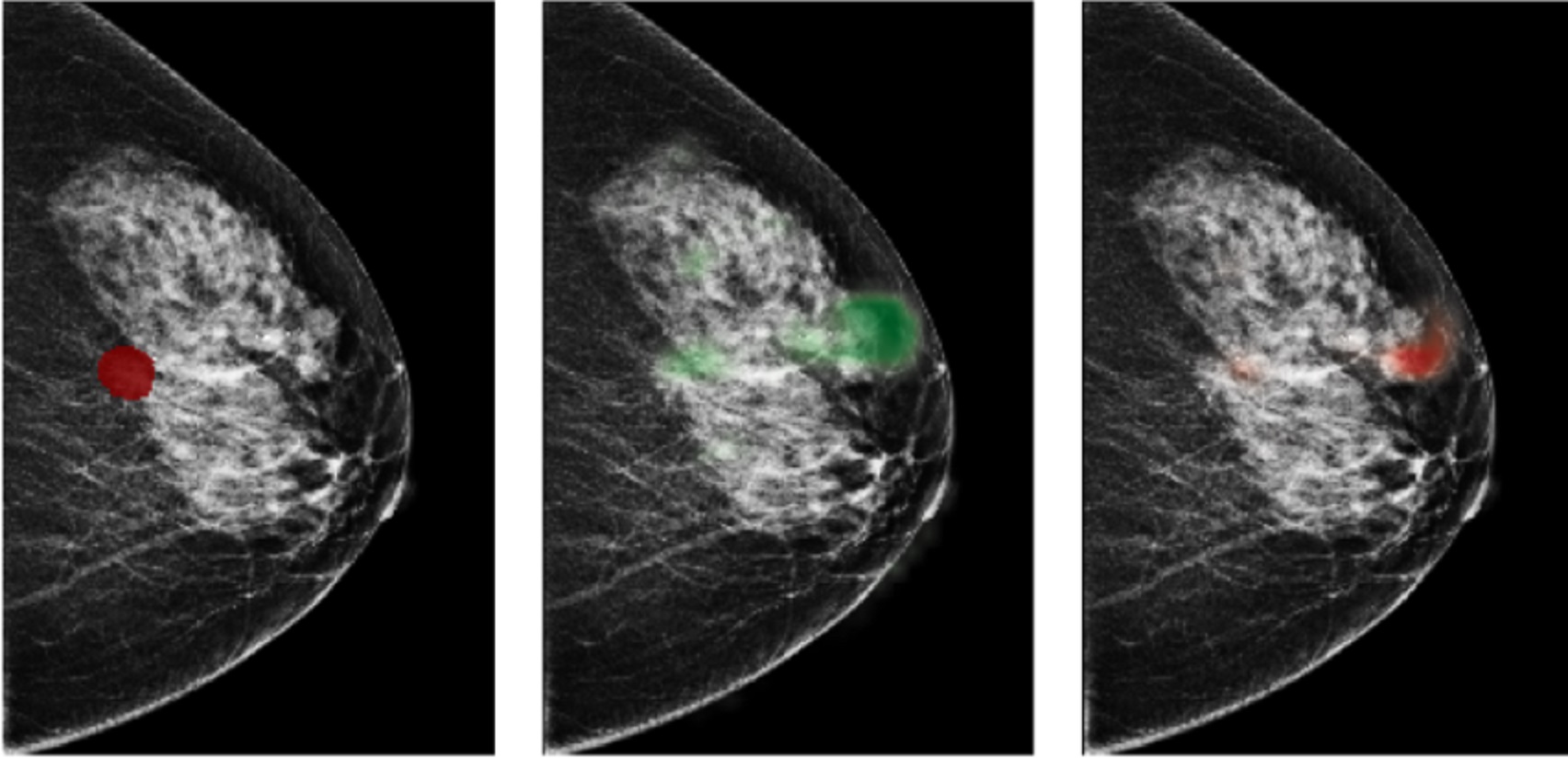
Discover how deep learning technologies revolutionize breast cancer screening, efficiently identifying false positives while ensuring the detection of true cancer cases. Through rigorous evaluation and validation, these AI-driven tools demonstrate significant reductions in unnecessary callbacks and biopsies, optimizing workflow efficiency and enhancing patient experience. The findings underscore the transformative potential of AI in healthcare, paving the way for enhanced diagnostic accuracy and improved outcomes in breast cancer screening and beyond.
In the realm of breast cancer screening, the interpretation of mammograms poses challenges for clinicians, often leading to false positives and unnecessary medical interventions. To address this issue, researchers have harnessed the power of deep learning, developing innovative tools to mitigate false positives while preserving the detection of true cancer cases. This introduction sets the stage for exploring the efficacy of deep learning technologies in revolutionizing breast cancer screening, highlighting their potential to streamline workflow efficiency and enhance patient care.
Screening mammography plays a pivotal role in the early detection of breast cancer, significantly impacting patient outcomes. However, the interpretation of mammograms can be challenging for radiologists, often resulting in false positives. These false positives not only cause unnecessary anxiety for patients but also strain medical resources. To address this issue, researchers have developed a deep learning tool aimed at reducing false positives without compromising the detection of true cancer cases.
The Role of Artificial Intelligence in Breast Cancer Screening
Artificial intelligence (AI) has emerged as a promising solution to improve the accuracy and efficiency of breast cancer screening. By leveraging AI-enabled tools, radiologists can streamline their workflow by identifying and removing low-risk mammograms, allowing them to focus their attention on images that exhibit suspicious characteristics indicative of breast cancer. However, the successful implementation of AI in this context hinges on its ability to differentiate between false positives and true cancer cases accurately.
Evaluating the Deep Learning Tool
In a recent study published in Radiology: Artificial Intelligence, researchers evaluated a deep learning model developed by technology startup Whiterabbit.ai. The goal was to assess its effectiveness in reducing false positives in screening mammography while ensuring the detection of true cancer cases. The study involved a simulation study followed by validation using real patient data from multiple datasets in the United States and the United Kingdom.
Study Methodology
The research team trained the deep learning tool on a comprehensive dataset consisting of 2D digital mammograms, including both normal cases and those containing cancer. This dataset, comprising 123,248 mammograms, was meticulously curated and evaluated by radiologists from Washington University. Following the training phase, the model underwent validation using independent datasets from various healthcare institutions.
Results and Implications
The findings of the study revealed promising results regarding the efficacy of the deep learning tool in reducing false positives without missing true cancer cases. Across all datasets, the utilization of the AI-driven tool led to significant reductions in unnecessary diagnostic callbacks and benign needle biopsies. For instance, in the first US dataset, the model reduced screening examinations requiring radiologist interpretation by 41.6 percent and diagnostic examination callbacks by 31.1 percent, while also decreasing benign needle biopsies by 7.4 percent.
Significance of the Findings
The study underscores the potential of AI-driven tools to alleviate the burden on clinicians while maintaining high standards of care in breast cancer screening. By accurately identifying low-risk mammograms, AI can assist radiologists in focusing their expertise on cases that warrant further evaluation, thereby optimizing workflow efficiency and resource allocation. Furthermore, the reduction in false positives enhances patient experience by minimizing unnecessary anxiety and medical interventions.
Future Directions and Concluding Remarks
The successful application of deep learning in reducing false positives in screening mammography sets a promising precedent for the integration of AI-driven technologies in healthcare. Moving forward, continued research and development in this field are essential to further refine and validate these tools for widespread clinical use. As AI continues to evolve, its role as a supportive tool in medical imaging analytics will become increasingly integral, empowering healthcare professionals to deliver more accurate and efficient care to patients.
Beyond Breast Cancer Screening: Expanding the Scope of AI in Healthcare
The study’s findings exemplify the broader potential of AI in medical imaging analytics beyond breast cancer screening. Recent advancements, such as the development of AI-based video biomarkers like the Digital Aortic Stenosis Severity Index (DASSi), highlight the versatility of AI in identifying and stratifying risks associated with various medical conditions. By leveraging AI-driven technologies, clinicians can enhance their diagnostic capabilities and improve patient outcomes across diverse healthcare domains.