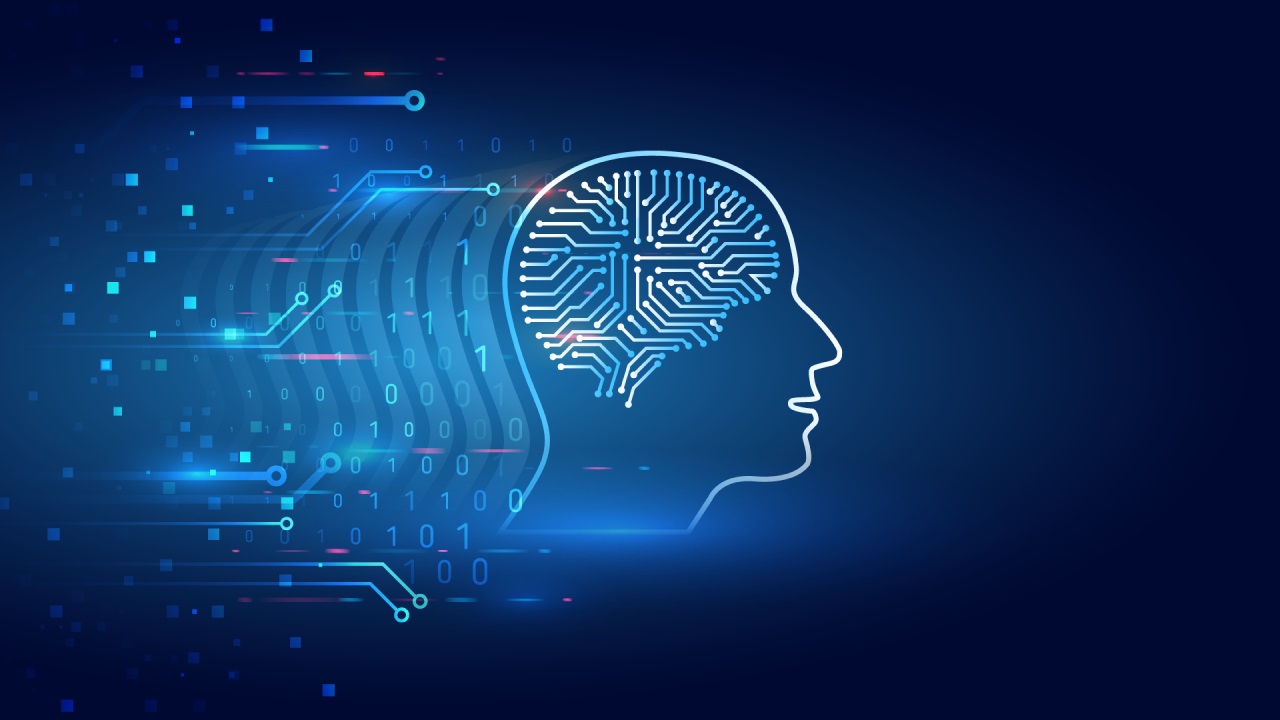
BeatProfiler, a novel suite of cardiac analysis tools powered by machine learning, marks a groundbreaking advancement in cardiac research. Developed by Columbia University researchers, BeatProfiler automates heart cell analysis, facilitating precise measurements of contractility and other crucial indicators of cardiac function. By streamlining analyses and minimizing errors, BeatProfiler promises to accelerate drug discovery and enhance diagnostic accuracy in cardiac diseases. Its open-source nature fosters collaboration and innovation within the scientific community, positioning it as a transformative tool poised to reshape cardiovascular research and clinical practice.
In the landscape of cardiac research, understanding heart function and testing new drugs remain complex and time-intensive endeavors. Columbia University researchers address these challenges with BeatProfiler, an innovative suite of cardiac analysis tools fueled by machine learning. By automating heart cell analysis and enabling precise measurements of contractility and calcium handling, BeatProfiler streamlines analyses and enhances efficiency in drug discovery and disease diagnosis. This introduction outlines the necessity for such advancements and sets the stage for the transformative impact of BeatProfiler in revolutionizing cardiac research and clinical care.
A Breakthrough in Cardiac Research: Introducing BeatProfiler
Columbia University’s Innovative Approach to Cardiac Function Assessment and Drug Response Prediction
In the ever-evolving landscape of cardiac research, the integration of machine learning has emerged as a pivotal tool, enabling a deeper understanding of cardiac function and revolutionizing drug response prediction. Recently unveiled by Columbia University researchers and published in the IEEE Open Journal of Engineering in Medicine and Biology, a groundbreaking study introduces a novel approach poised to redefine how we assess cardiac health and tailor drug interventions.
The intricacies of heart function evaluation and the testing of novel pharmaceuticals to combat cardiac diseases pose formidable challenges, characterized by their complexity and time-intensive nature. While advancements in cellular and engineered tissue models have alleviated some of these challenges, significant hurdles persist.
Central to understanding cardiac physiology are the assessments of contractile response and calcium handling. While methodologies exist for testing these parameters in cellular and tissue models, they are often plagued by inefficiencies, susceptibility to errors, and reliance on specialized equipment. Recognizing these limitations, the Columbia University research team embarked on a mission to develop an innovative approach capable of accurately quantifying contractile function, calcium handling, and force generation across in vitro cardiac models, thereby facilitating comprehensive analyses of cardiac function and drug response.
Enter BeatProfiler – a groundbreaking suite of cardiac analysis tools empowered by machine learning. Designed to automate heart cell analysis using video data, BeatProfiler represents a paradigm shift in cardiac research by enabling precise measurements of contractility and other crucial indicators of cardiac function. By amalgamating multiple tools, this innovative system streamlines analyses, significantly enhancing efficiency while minimizing the risk of errors.
Project leader Gordana Vunjak-Novakovic, PhD, lauds BeatProfiler as a transformative tool, emphasizing its speed, comprehensiveness, automation, and cross-platform compatibility. This accessibility ensures that investigators and clinicians alike can leverage its capabilities seamlessly.
The genesis of BeatProfiler stems from the pressing clinical imperative to diagnose heart diseases accurately and optimize treatment modalities. Crucially, this necessitates the ability to evaluate the efficacy of potential therapeutics on in vitro cardiac models. BeatProfiler is poised to catalyze these endeavors by empowering research teams to quantify the function of cardiomyocytes, pivotal for investigations spanning genetic cardiomyopathies, immune-mediated inflammation, cosmic radiation, and drug discovery.
In comparative assessments, BeatProfiler has demonstrated superiority over existing tools, furnishing precise and reliable analyses of cardiomyocyte function without sacrificing efficiency. Notably, the suite of tools exhibits the remarkable ability to discern subtle shifts in engineered cardiac tissue force response, outpacing existing methodologies by up to 50 times in certain scenarios.
The unparalleled performance of BeatProfiler enables researchers to delineate between various diseases and severity levels swiftly while expeditiously evaluating drugs’ impacts on heart function. Lead author Youngbin Kim underscores the significance of machine learning in enabling such advanced functional measurements, facilitating the accurate classification of cardiac drugs based on their effects on the heart.
Moreover, BeatProfiler’s open-source nature renders it freely accessible to researchers, fostering collaboration and innovation within the scientific community. Looking ahead, the Columbia University team is committed to further validating and expanding BeatProfiler for diverse cardiac research applications. Ultimately, they envision adapting this revolutionary tool for pharmaceutical settings to accelerate candidate drug testing, thus hastening the translation of research insights into clinical practice.
Harnessing Artificial Intelligence for Cardiovascular Advancements
Beyond the realm of cardiac function assessment, artificial intelligence (AI) continues to spearhead transformative innovations in cardiovascular research. A recent milestone achieved by a multi-institutional research consortium introduces the Digital Aortic Stenosis Severity Index (DASSi), an AI-based video biomarker poised to enhance clinicians’ prognostic capabilities concerning aortic stenosis development and progression.
Utilizing echocardiographic signatures, DASSi exhibits remarkable performance in stratifying the risk of aortic stenosis across diverse patient cohorts, including those without the condition and individuals with mild or moderate forms of the ailment at baseline. This breakthrough underscores the burgeoning role of AI in unraveling complex cardiovascular pathologies, heralding a new era of precision medicine and personalized care delivery.
The convergence of machine learning and cardiac research heralds a new era of precision medicine and personalized care delivery. BeatProfiler, with its unparalleled ability to automate heart cell analysis and discern subtle shifts in cardiac function, stands as a beacon of innovation in cardiovascular research. By accelerating drug discovery and enhancing diagnostic accuracy, BeatProfiler promises to optimize patient outcomes and reshape the landscape of cardiac care. Moving forward, continued validation and expansion of BeatProfiler hold the potential to further revolutionize cardiac research, fostering proactive disease management and tailored therapeutic interventions.