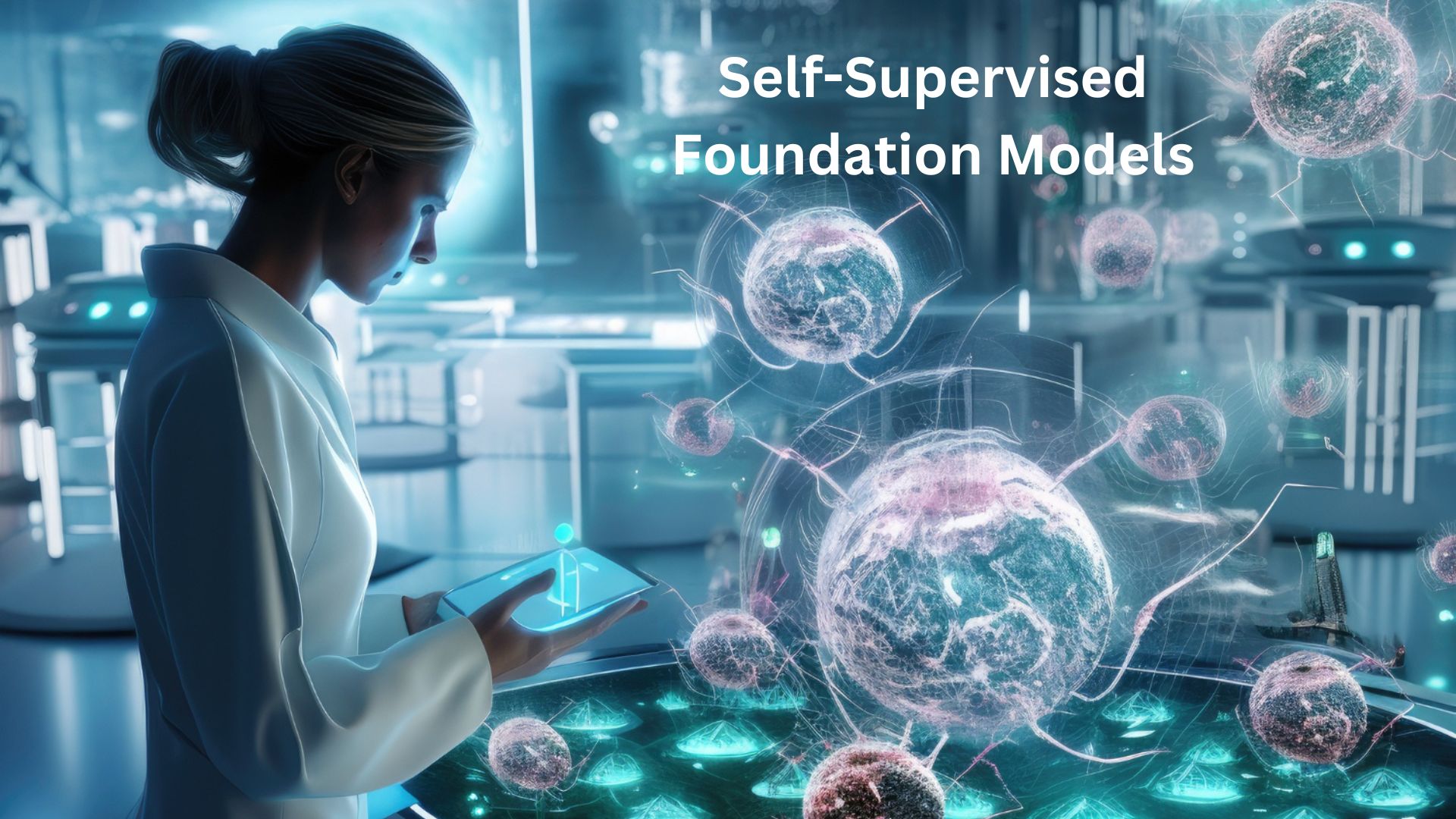
Table of contents:
- Introduction
- Addressing Disparities in Computational Pathology 2.1.
- Limitations of Current AI Tools:
- Quantifying Performance Disparities:
- Methodology and Findings:
- Bias Mitigation Techniques:
- Leveraging Self-supervised Vision Foundation Models:
- Conclusion and Future Directions:
- Call to Action
- FAQs
Self-supervised Foundation Models: Bridging Bias Gaps in Histopathology AI
Introduction:
Disparities in Image Classification Models
There exists a concerning discrepancy in the accuracy of image classification models, with notably higher performance rates observed in white patients compared to Black patients. However, there is hope that foundation models could serve as a remedy to bridge these disparities.
Addressing Disparities in Computational Pathology:
Acknowledging Variation in Computational Pathology Models
Researchers from Mass General Brigham have unearthed noteworthy variations in the performance of standard computational pathology models across different demographic groups. Encouragingly, their recent study published in Nature Medicine suggests that foundation models hold promise in partially mitigating these disparities.
Limitations of Current AI Tools:
Challenges Hindering Effective AI Utilization in Pathology
The effective deployment of AI tools in pathology faces significant impediments, chiefly stemming from the inadequate representation of minoritized patient populations in training datasets. Such shortcomings underscore the pressing health equity concerns intertwined with AI applications in healthcare.
Quantifying Performance Disparities:
Unveiling and Addressing Performance Disparities
To confront these challenges head-on, the research team embarked on a mission to quantify and alleviate the performance discrepancies exhibited by computational pathology models across diverse demographic groups through bias mitigation techniques.
Methodology and Findings:
Insights from Comprehensive Data Analysis
Utilizing datasets from the Cancer Genome Atlas and the EBRAINS brain tumor atlas, predominantly comprising data from white patients, the researchers developed computational pathology systems for various cancer subtyping tasks. Subsequent testing on histology slides from a cohort of over 4,300 cancer patients unveiled glaring performance gaps, particularly favoring white patients across multiple cancer subtyping tasks.
Bias Mitigation Techniques:
Employing Strategies to Counteract Biases
In response to these disparities, the research team deployed machine learning-based bias mitigation strategies, including prioritizing examples from underrepresented populations during model training. Despite modest reductions in observed biases, substantial disparities persisted.
Leveraging Self-supervised Vision Foundation Models:
Exploring Innovative Approaches for Bias Reduction
Building upon these efforts, researchers investigated the potential of self-supervised vision foundation models—AI tools trained on expansive datasets—to further diminish performance gaps. By extracting richer feature representations from histology images, these foundation models demonstrated significant enhancements in performance.
Conclusion and Future Directions:
Addressing Persistent Disparities and Charting the Path Ahead
Although progress has been made, significant performance disparities persist across demographic groups, underscoring the imperative for continued model refinement. Future endeavors will focus on exploring multi-modality foundation models, integrating diverse data sources like genomics and electronic health records, to surmount existing obstacles and foster equitable healthcare outcomes.
Call to Action
The findings from this study represent a call to action for developing more equitable AI models in medicine. It emphasizes the need for scientists to use more diverse datasets in research and for regulatory and policy agencies to include demographic-stratified evaluations of these models in their assessment guidelines before approving and deploying them, to ensure that AI systems benefit all patient groups equitably.
Advancing Health Equity through AI
These efforts are the latest to investigate how AI could advance health equity, including projects like the “Trustworthy AI to Address Health Disparities in Under-resourced Communities” (AI-FOR-U), which aims to develop explainable, fair risk prediction models to tackle disparities in healthcare.
FAQs
1. Why do image classification models perform differently across demographic groups in pathology AI?
– This FAQ addresses the root cause of performance disparities in pathology AI models, exploring factors such as the underrepresentation of certain demographic groups in training data and inherent biases in algorithmic decision-making.
2. How do self-supervised foundation models mitigate bias in histopathology AI?
– This question delves into the specific mechanisms and techniques employed by self-supervised vision foundation models to reduce bias in histopathology AI, offering insights into how richer feature representations contribute to improved performance across diverse patient populations.
3. What are the implications of the study’s findings for the future of AI in healthcare?
– This FAQ explores the broader significance of the study’s results, discussing the implications for developing more equitable AI models in medicine, the importance of diverse datasets in research, and the role of regulatory and policy agencies in ensuring AI systems benefit all patient groups equitably.