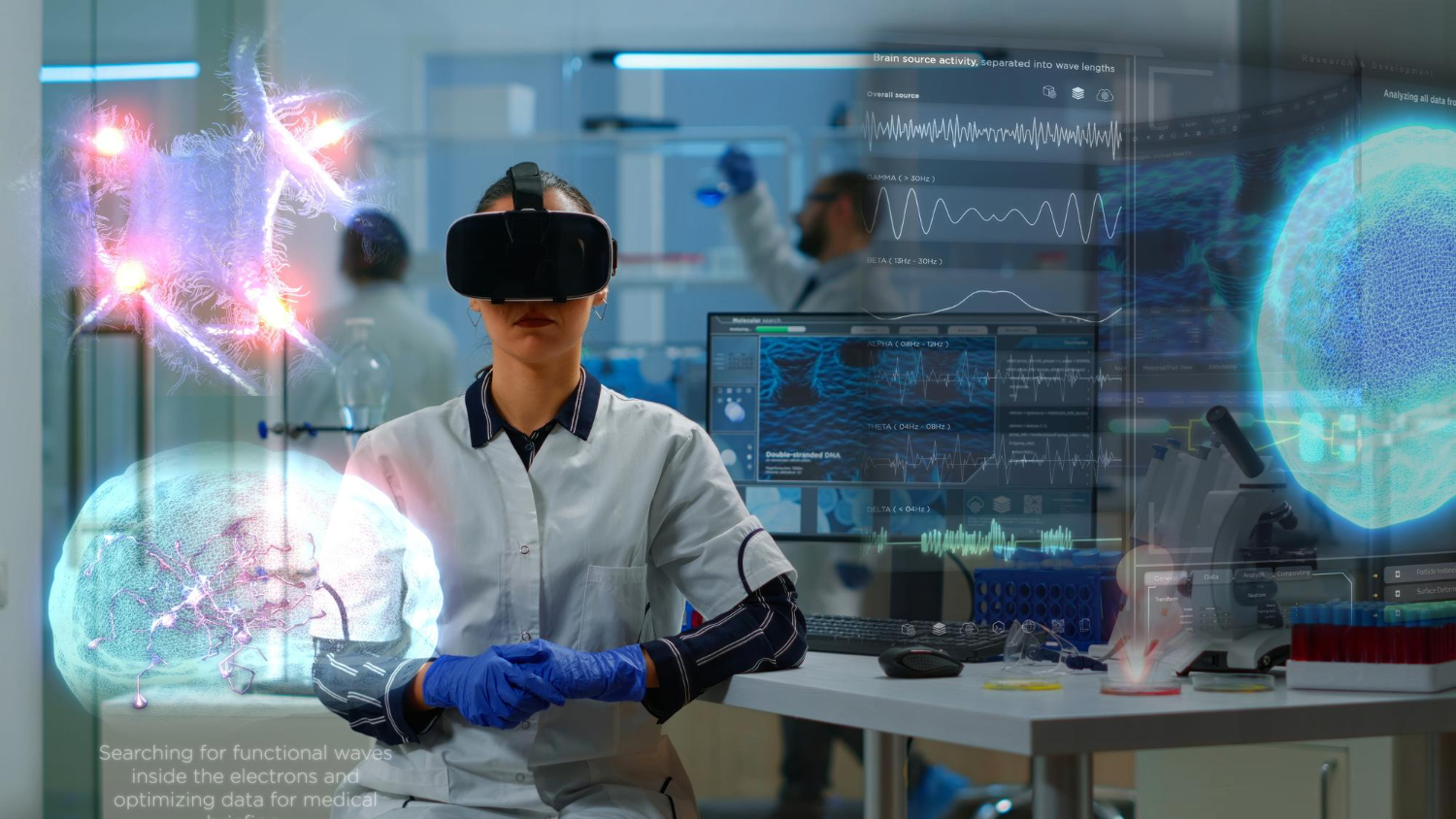
Table of Contents
- Introduction
- Understanding Common Variable Immunodeficiency (CVID)
- Challenges in Diagnosing CVID
- The Role of Machine Learning in Diagnosing CVID
- PheNet: A New Diagnostic Machine Learning Model
- Implications and Future Directions
- FAQs
Introduction
Recent advancements in machine learning have provided significant opportunities to enhance healthcare diagnostics. A prime example is the development of a model by researchers from the University of California, Los Angeles (UCLA) Health, which leverages Electronic Health Record (EHR) data to identify patients likely suffering from undiagnosed immunodeficiency diseases, specifically Common Variable Immunodeficiency (CVID).
Understanding Common Variable Immunodeficiency (CVID)
What is CVID?
CVID is one of the most frequent types of inborn errors of immunity (IEI), comprising a group of about 500 inherited conditions that cause immune system dysfunction. It affects approximately one in every 25,000 people and is characterized by antibody deficiencies and an impaired immune response.
Genetic Complexity of CVID
Unlike conditions caused by mutations in a single gene, CVID can involve mutations in any of over 60 different genes. This genetic diversity makes it difficult to diagnose through traditional genetic testing, contributing to the challenges in its identification and management.
Challenges in Diagnosing CVID
Delays in Diagnosis
Patients with rare diseases like CVID often experience significant delays in diagnosis. These delays are exacerbated by the rarity and varied symptoms of the condition, which often overlap with more common illnesses, leading to unnecessary tests and extended suffering.
Fragmentation of Care
CVID symptoms can intersect with several medical specialties, from ear, nose, and throat clinics to pulmonology. This fragmentation across specialties often results in further delays in diagnosis as comprehensive knowledge about immune deficiencies is not widespread among specialists.
The Role of Machine Learning in Diagnosing CVID
Revolutionizing Healthcare with AI
Machine learning and artificial intelligence are increasingly integrating into healthcare, offering new methodologies for processing complex and vast datasets. By analyzing patterns in EHR data, these technologies can help speed up the diagnosis of elusive diseases like CVID.
PheNet: A New Diagnostic Machine Learning Model
Development and Functionality
The UCLA research team developed PheNet, a machine-learning model that infers disease signatures from existing patient records and relevant medical literature. By analyzing these data, PheNet assigns a numerical score to patients, ranking their likelihood of having CVID.
Validation and Results
PheNet was tested and validated on large datasets, including 6 million patient records from multiple sources. The model successfully flagged a significant number of patients who were later confirmed to have CVID, demonstrating its potential to reduce diagnostic delays significantly.
Implications and Future Directions
Expanding to Other Rare Diseases
The success of PheNet with CVID opens the door to its application to other rare diseases, potentially transforming diagnostic processes across various conditions. The UCLA team is already working on enhancing the model’s precision and expanding its reach.
Implementation Across Medical Centers
Efforts are underway to implement PheNet across all University of California medical centers. This widespread use is expected to facilitate earlier diagnosis and better health outcomes for many patients.
FAQs
Q1: What is Common Variable Immunodeficiency (CVID)?
A1: CVID is a type of immune disorder characterized by antibody deficiencies and an impaired immune response, affecting roughly one in every 25,000 people.
Q2: How does the PheNet model work?
A2: PheNet uses machine learning to analyze EHR data and medical literature to identify patterns that might indicate a patient has CVID, assigning a likelihood score based on these patterns.
Q3: What are the benefits of diagnosing CVID early?
A3: Early diagnosis can prevent unnecessary testing, reduce psychological and financial stress, and improve treatment effectiveness, leading to better overall health outcomes.
Q4: Can PheNet be used for other diseases?
A4: Yes, the research team plans to adapt PheNet for other rare diseases, aiming to enhance its diagnostic capabilities and precision further.
This innovative approach not only underscores the potential of machine learning in healthcare but also highlights the critical need for technological integration to address diagnostic challenges in rare diseases like CVID.