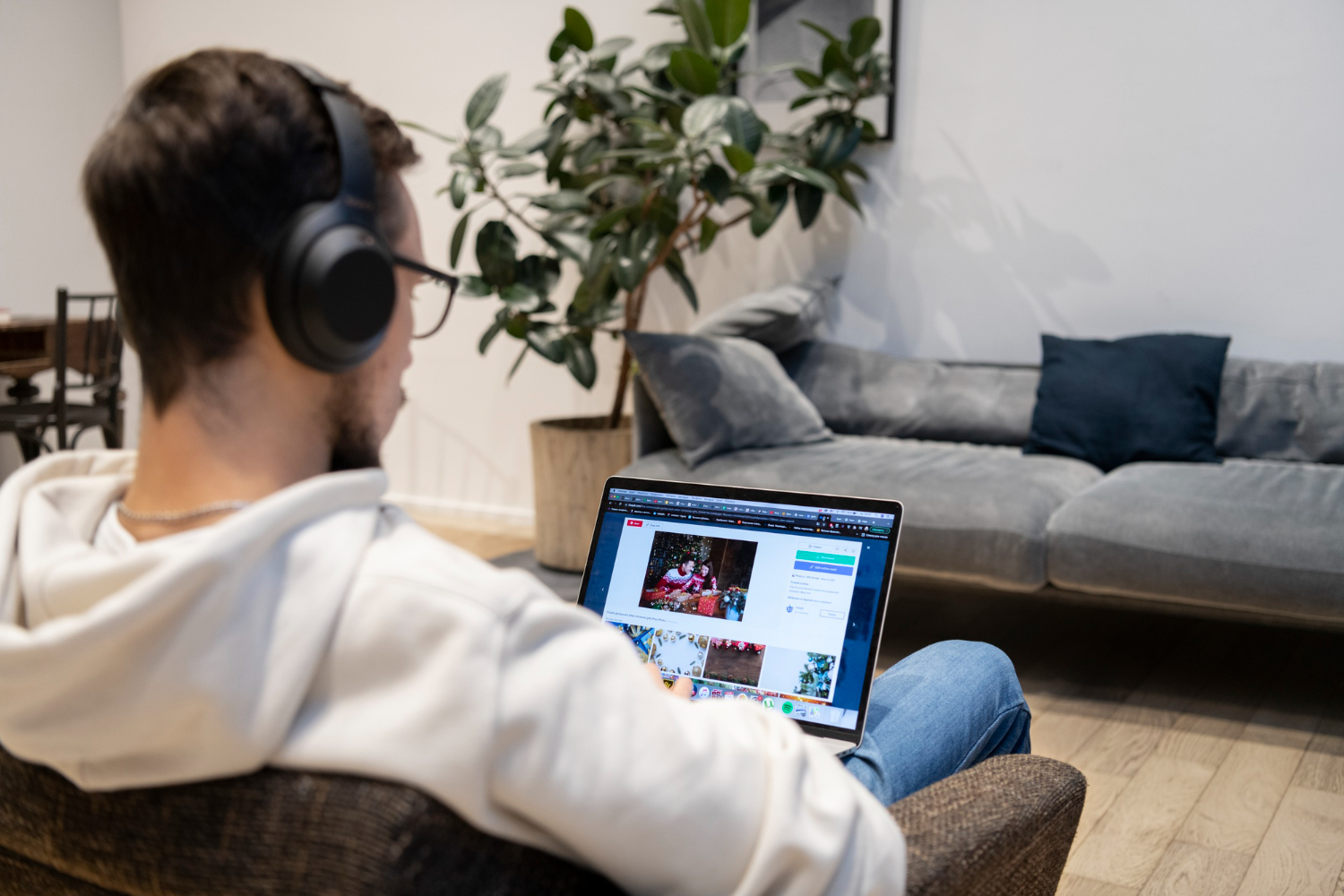
Table of Contents
- Introduction
- The Growing Need for Advanced Mental Health Monitoring
- The Evolution of AI in Mental Health Research
- Study Insights and Achievements
- Challenges in Utilizing Social Media Data
- The Future of AI in Mental Health Monitoring
- FAQs
- Conclusion
Introduction
In an era where mental health increasingly dictates community well-being, researchers are turning to innovative technologies to enhance understanding and monitoring. Artificial Intelligence (AI), combined with vast data from social media platforms, presents a novel opportunity to observe and analyze mental health trends at the population level.
The Growing Need for Advanced Mental Health Monitoring
The Role of Mental Health in Public Health
Mental health issues such as depression and anxiety are significant contributors to global morbidity, influencing outcomes like suicide and substance abuse. This underscores the urgent need for effective monitoring and intervention strategies.
Limitations of Traditional Assessment Methods
Traditional mental health assessments often rely on self-reported data through phone surveys, which can be costly and yield limited data. These methods face challenges in scalability and timeliness, which are critical in addressing the dynamic nature of mental health crises.
The Evolution of AI in Mental Health Research
Development of the AI Framework
Researchers from Stony Brook, Stanford, and the University of Pennsylvania have pioneered an AI system that harnesses social media data to monitor mental health. This system uses language-based cues to gauge emotional and psychological states.
Mechanisms of Language-Based Assessments
The AI framework employs natural language processing techniques to analyze the frequency and context of words related to emotional distress, providing a nuanced understanding of community mental health levels.
Study Insights and Achievements
Methodology and Data Analysis
The study analyzed nearly one billion social media posts from over two million users across 1,418 U.S. counties. This large-scale analysis allows for an unprecedented mapping of mental health across diverse populations.
Accuracy and Reliability
Results show that AI-derived data aligns closely with results from traditional surveys, often exceeding them in predictive accuracy and reliability, especially when adjusted for socio-demographic factors.
Challenges in Utilizing Social Media Data
Ethical Considerations
The use of social media data for research purposes raises important ethical questions regarding privacy and consent. Researchers must navigate these issues carefully to maintain trust and integrity in their methodologies.
Data Accessibility and Policy Changes
The dynamic nature of social media platforms, including changes in user behavior, policies, and data access, poses challenges to the consistency and reliability of research data.
The Future of AI in Mental Health Monitoring
Potential Developments
Ongoing advancements in AI and machine learning promise to refine the accuracy and effectiveness of mental health assessments, potentially allowing for real-time monitoring and intervention.
Integration with Traditional Methods
Combining AI-driven techniques with conventional survey methods could lead to more robust mental health monitoring systems, helping to overcome biases and limitations inherent in each approach.
FAQs
1. What does AI identify in social media posts to assess mental health?
AI analyzes patterns of language use, such as word frequency and sentiment, to infer the mental state of users.
2. How can AI improve public health responses to mental health crises?
AI can provide rapid, large-scale assessments of mental health trends, helping policymakers and health professionals develop timely and targeted interventions.
3. What are the privacy implications of using AI in mental health research?
Using AI to analyze social media must balance the need for valuable health insights with the protection of individual privacy, adhering to ethical standards and regulations.
Conclusion
The integration of AI with social media data offers profound possibilities for advancing our understanding and management of mental health on a population scale. This approach not only enhances the precision and breadth of research but also provides a foundation for more responsive and effective public health strategies.