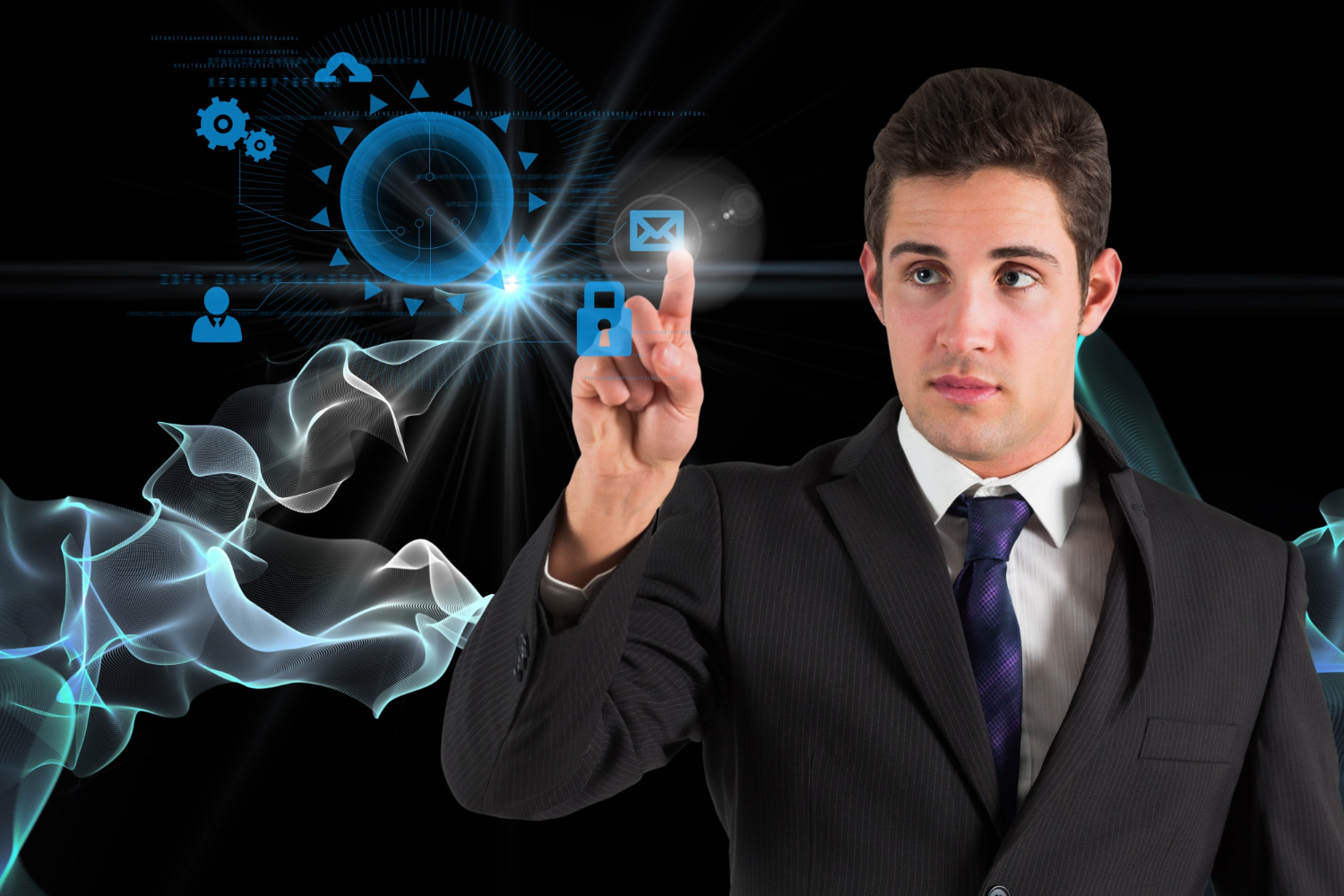
Introduction
The Dana-Farber Cancer Institute (DFCI) has taken significant strides in integrating large language models (LLMs) into its operations, focusing on non-clinical applications. This initiative, led by Renato Umeton, the Director of AI Operations and Data Science Services, aims to enhance clinical and basic research as well as operational tasks while ensuring compliance with stringent security and privacy standards.
Understanding Large Language Models (LLMs)
LLMs, such as GPT-4, are advanced AI tools capable of understanding and generating human-like text. These models hold immense potential in various sectors, including healthcare, by automating repetitive tasks, extracting information, and supporting decision-making processes.
Integrating LLMs at Dana-Farber Cancer Institute
Governance and Ethical Considerations
The integration of LLMs at DFCI required addressing multiple governance and ethical challenges. Establishing a comprehensive AI governance framework was essential. This framework involved forming a multidisciplinary AI Governance Committee, which included legal, clinical, research, technical, and bioethics experts, as well as patient representatives.
Technical and Regulatory Challenges
Deploying LLMs in healthcare comes with significant technical and regulatory hurdles. DFCI navigated these challenges by developing a secure API, ensuring HIPAA compliance, and creating a private exploratory environment to evaluate, test, and deploy LLMs for non-clinical purposes.
Best Practices for Secure LLM Deployment
Training and Upskilling Workforce
One of the critical success factors in the deployment of LLMs at DFCI was the emphasis on workforce training. Employees were trained on the proper and secure use of LLMs, with reskilling and upskilling initiatives to increase adoption and proficiency.
Secure API Development
To embed AI into their software applications securely, DFCI developed a robust and secure API. This API enabled developers to integrate AI capabilities into their applications while maintaining stringent security protocols.
Case Study: GPT4DFCI
Implementation and Use Cases
GPT4DFCI, a secure and HIPAA-compliant generative AI tool based on GPT-4 models, is at the core of DFCI’s AI operations. This tool assists in various non-clinical tasks, such as extracting information from notes and reports, automating repetitive tasks, and streamlining administrative documentation.
Security and Compliance Measures
The implementation of GPT4DFCI involved multiple layers of security. The innermost layer uses supporting AI models to filter harmful content. The next layer logs all user activities for auditing purposes. The outermost layer provides a simple user interface with training materials and a support ticketing system.
Insights from Renato Umeton
Key Opportunities and Challenges
Renato Umeton highlights that while LLMs offer significant opportunities to improve efficiency and data management, they also present challenges in terms of ethics, legality, and regulatory compliance. The careful balance of innovation and patient safety is crucial.
Future Prospects
Looking ahead, Umeton envisions better data and AI leading to improved practices and patient outcomes. Sharing DFCI’s experiences aims to provide insights for other healthcare organizations considering similar AI deployments.
Conclusion
The Dana-Farber Cancer Institute’s journey in integrating secure LLMs offers valuable lessons in overcoming governance, ethical, regulatory, and technical challenges. By establishing a comprehensive AI governance framework and emphasizing workforce training, DFCI has successfully deployed LLMs for non-clinical applications, paving the way for future advancements in healthcare AI.
Discover the latest Provider news updates with a single click. Follow DistilINFO HospitalIT and stay ahead with updates. Join our community today!
FAQs
Q1: How is Dana-Farber Cancer Institute using LLMs?
A: Dana-Farber is using LLMs for non-clinical applications, including clinical and basic research and operational tasks. They have created a secure and private environment to test and deploy these models, explicitly excluding direct clinical care.
Q2: What are the main challenges Dana-Farber faced in integrating LLMs?
A: The main challenges included governance, ethical, regulatory, and technical issues. They needed to ensure the secure and compliant use of AI while addressing potential risks and ethical concerns.
Q3: How did Dana-Farber ensure the secure use of LLMs?
A: Dana-Farber deployed a secure API, trained their workforce on proper LLM use, and implemented supporting AI models to filter dangerous content. They also established a comprehensive logging and auditing system to monitor usage.
Q4: What are the benefits of using LLMs in healthcare?
A: LLMs can improve efficiency in healthcare by automating tasks, enhancing data analysis, and streamlining documentation. In the long term, they can lead to better practices and improved patient outcomes.
Q5: What can other healthcare organizations learn from Dana-Farber’s experience?
A: Other organizations can learn the importance of establishing a robust AI governance framework, phased and controlled AI rollouts, comprehensive training for users, and the careful balancing of innovation with patient safety and data privacy.
Leave a Reply