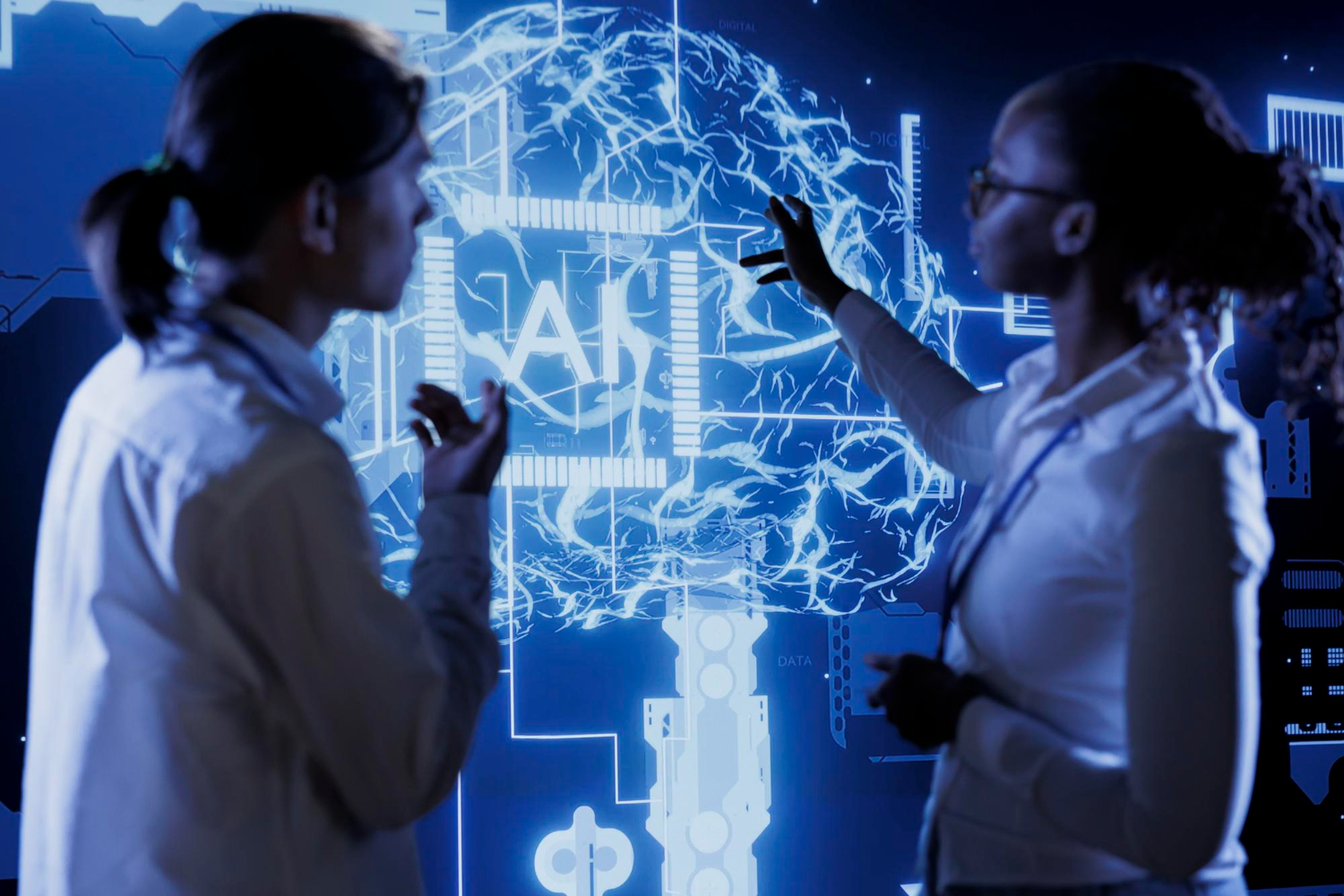
Leverage AI Technology to Transform Healthcare Outcomes
Artificial intelligence (AI) and machine learning (ML) are transforming industries, particularly healthcare. As organizations adopt AI, they aim to streamline operations, improve patient outcomes, and innovate care delivery. However, successful AI implementation requires a well-planned strategy. This article, inspired by insights from Alex MacLeod, Director of Healthcare Solution Innovation at InterSystems, outlines steps to ensure healthcare organizations can best leverage AI, including generative artificial intelligence (GenAI), to maximize benefits.
Introduction to AI in Healthcare
The rapid advancement of AI technology has opened new doors for healthcare organizations. From machine learning algorithms that detect patterns in medical data to generative AI that creates treatment plans, the potential applications are vast. AI’s ability to handle and analyze enormous amounts of data is particularly valuable in healthcare, where accurate and timely decisions are crucial.
Healthcare organizations that want to leverage AI effectively need to approach it strategically. By understanding the technology, identifying valuable use cases, and preparing the organization for change, AI can significantly improve patient care and operational efficiencies.
Steps to Effectively Leverage AI Technology
Identify Key Use Cases for AI
The first step in leveraging AI is identifying where it can provide the most value. Healthcare organizations should focus on areas where AI can improve outcomes or streamline processes. For instance, AI can enhance diagnostic accuracy, reduce administrative burdens, or support medical research. Leaders should prioritize high-impact use cases and pilot AI projects that align with their strategic goals.
Prepare Data Infrastructure
AI and machine learning systems require vast amounts of data to function effectively. Organizations must ensure their data infrastructure is robust enough to handle these demands. This includes ensuring data accuracy, integrating data from multiple sources, and establishing protocols for data privacy and security. AI systems rely on high-quality data, so investing in data management is critical for success.
Ensure Staff Training and Engagement
For AI to be successfully adopted, healthcare staff must be trained on how to use AI tools. This includes technical training and fostering a culture that embraces innovation. Involving staff early in the process helps overcome resistance to new technologies and ensures AI tools are used effectively in day-to-day operations. Training should also cover ethical considerations and how AI should complement human judgment, not replace it.
Partner with Technology Vendors
Partnering with experienced AI vendors is crucial. Many healthcare organizations lack the internal resources to develop AI solutions from scratch. Working with vendors that specialize in AI technology can help organizations adopt AI faster and more efficiently. These partnerships ensure AI solutions are tailored to the unique needs of the organization.
AI Use Cases in Healthcare
Improving Diagnostic Accuracy
AI tools, especially in areas like medical imaging, can significantly enhance diagnostic accuracy. Machine learning algorithms detect patterns that may be missed by human eyes, such as early-stage cancers or subtle changes in heart rhythms. AI also assists in the interpretation of CT scans, MRIs, and X-rays, helping radiologists make faster, more accurate diagnoses.
Streamlining Administrative Tasks
Administrative tasks, such as patient scheduling, billing, and claims processing, are time-consuming but necessary. AI can automate these processes, reducing the workload on administrative staff and minimizing human errors. For example, natural language processing (NLP) tools can read and interpret patient records, automating data entry and coding.
Personalizing Patient Care
AI can analyze patient data to identify patterns and recommend personalized treatment plans. By understanding patient histories and genetic profiles, AI tools can help clinicians tailor treatments to each individual. This personalized approach leads to better outcomes and more efficient use of resources, particularly in managing chronic conditions like diabetes or heart disease.
AI in Medical Research and Drug Development
AI is also transforming medical research. Machine learning algorithms process vast amounts of data from clinical trials, helping researchers identify trends and potential new treatments faster. In drug development, AI can analyze chemical structures and predict how new compounds might interact with human biology, speeding up the discovery of new medications.
Challenges in Adopting AI
Data Privacy and Security Concerns
With AI’s reliance on large datasets, data privacy and security are major concerns. Healthcare organizations must comply with regulations like HIPAA to ensure patient data is protected. Robust cybersecurity measures are essential to prevent data breaches, which could compromise patient trust and lead to regulatory penalties.
Integration with Existing Systems
AI tools must be seamlessly integrated into existing IT systems, including electronic health records (EHRs). This can be a challenge, as many legacy systems were not designed to work with AI technologies. Interoperability between AI tools and current systems is essential to prevent workflow disruptions.
Discover the latest Provider news updates with a single click. Follow DistilINFO HospitalIT and stay ahead with updates. Join our community today!
FAQs
1. How can AI improve patient care in healthcare organizations?
A. AI can enhance diagnostic accuracy, personalize treatment plans, and streamline administrative tasks, leading to better patient outcomes and operational efficiency.
2. What are the challenges healthcare organizations face when adopting AI?
A. Key challenges include data privacy and security concerns, integration with existing systems, and ensuring staff are trained and engaged with the technology.
3. Why is data quality important for AI in healthcare?
A. AI systems rely on high-quality data to make accurate predictions and analyses. Poor data quality can lead to inaccurate results and potentially harm patient outcomes.
4. How can organizations ensure successful AI adoption?
A. Organizations should identify key use cases, invest in data infrastructure, ensure staff training, and partner with experienced technology vendors to ensure successful AI adoption.