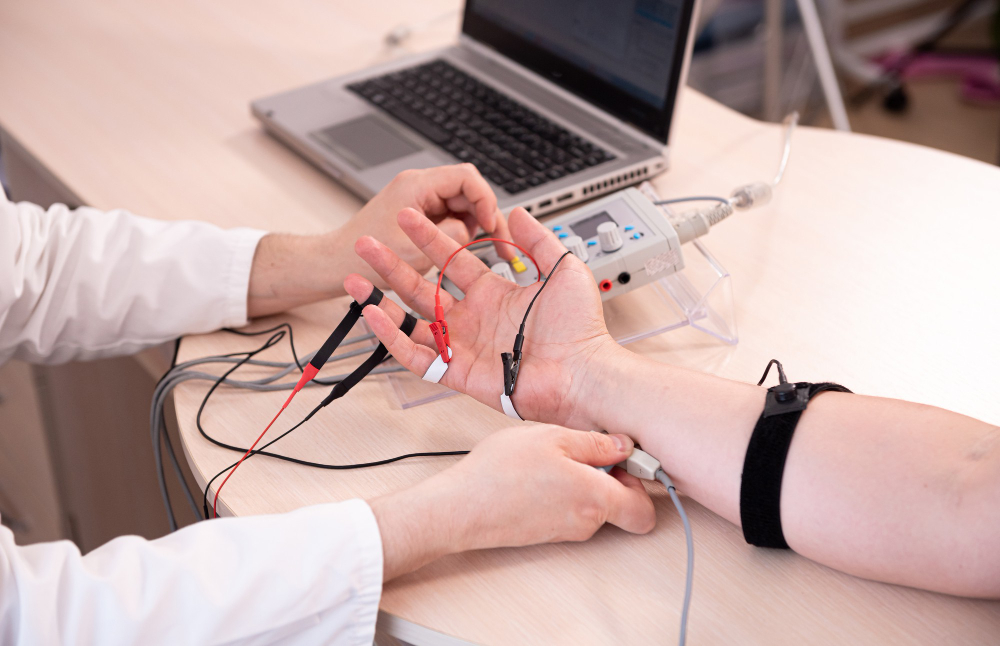
Researchers from Boston University have developed a machine learning model to provide personalized hypertension treatment recommendations. The model analyzes patient-specific characteristics and medical history to generate a customized hypertension prescription, suggesting the most appropriate medication for each individual. In a study using de-identified data from over 42,000 patients, the model outperformed existing algorithms and achieved a significant reduction in systolic blood pressure. This AI-driven approach has the potential to improve care quality and enable personalized medicine for underrepresented populations.
Boston University (BU) researchers have collaborated on the development of a machine learning (ML) model that aims to offer personalized hypertension treatment recommendations to clinicians, assisting them in selecting the most suitable medication for their patients. The study, recently published in BMC Medical Informatics and Decision Making, highlights the challenge of prescribing the most effective medication for patients with hypertension due to various factors like genetic traits and comorbidities that influence treatment response.
While personalized hypertension treatment holds promise in overcoming these obstacles, its adoption in clinical care has been limited. To address this, the BU researchers created an ML-based clinical decision support tool that provides real-time hypertension treatment suggestions to care teams, aiming to achieve more effective systolic blood pressure reduction compared to current standard of care methods.
The ML model utilizes patient-specific information, including medical history, demographics, vital signs, and electronic health record (EHR) test results, to generate customized hypertension prescriptions for clinicians. This novel algorithm leverages information from EHRs, exemplifying the power of AI in healthcare. Instead of merely predicting outcomes, the data-driven model suggests the most appropriate medication for each individual patient.
By analyzing an individual’s profile, the model generates a list of recommended medications along with the corresponding probability of success based on the patient’s data and the medication’s effectiveness in similar patient groups. The objective is to facilitate a personalized approach to hypertension treatment, maximizing the effectiveness of antihypertensive medications at an individual level.
To develop the model, the researchers utilized de-identified data from the medical records of 42,752 patients at Boston Medical Center (BMC) between 2012 and 2020. Patients were categorized into groups based on relevant characteristics such as demographics, medical history, and previous blood pressure readings. This data was then fed into the ML model, along with three additional algorithms designed to predict appropriate hypertension treatment plans.
Comparisons were made between the effectiveness of each model and the current standard of care. The ML model outperformed the second-best algorithm by 7.08% and demonstrated a 70.3% greater reduction in systolic blood pressure compared to the standard of care.
Furthermore, the ML model shed light on the value of reducing or discontinuing antihypertensive drugs for patients already taking multiple medications.
The study emphasized the potential of advanced predictive analytics in enhancing clinician decision-making and improving the quality of care, ultimately benefiting patient outcomes. By demonstrating that these models outperform the standard of care, they have the potential to assist healthcare professionals in delivering better treatment.
Moreover, the research highlighted how artificial intelligence (AI) models can contribute to improving care quality and personalized medicine approaches. By utilizing data from Boston Medical Center’s diverse patient population, this model opens up opportunities for tailoring care for underrepresented populations, providing individualized recommendations to enhance outcomes for these patients. This approach to personalized medicine has the potential to serve populations that have been underrepresented in national studies or overlooked during guideline development processes.