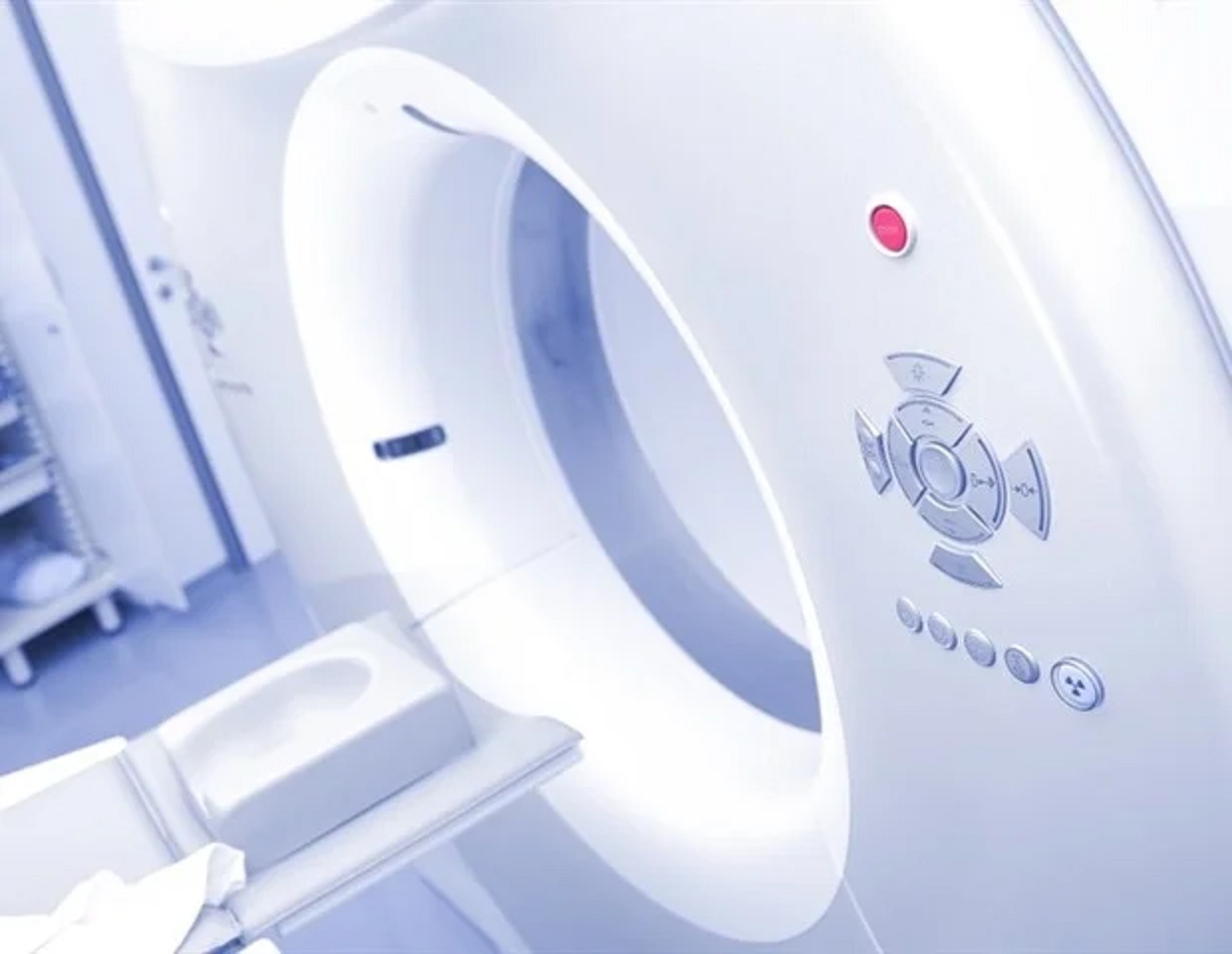
AI utilizes low-dose CT scans to improve risk prediction for lung cancer, cardiovascular disease, and mortality. AI-derived body composition measurements enhance risk assessment and allow early intervention for high-risk individuals. Opportunistic screening with CT scans offers valuable information about various health conditions. The study emphasizes the potential for routine clinical use and highlights the significance of myosteatosis in predicting health outcomes. Future research will explore longitudinal studies to monitor changes in body composition and their impact on health.
A study published in the Radiology Journal of the Radiological Society of North America (RSNA) reveals that Artificial Intelligence (AI) can utilize data from low-dose CT scans of the lungs to enhance risk prediction for death caused by lung cancer, cardiovascular disease, and other conditions.
The U.S. Preventive Services Task Force recommends annual lung screening with low-dose CT (LDCT) for people aged 50 to 80 years with a high risk of lung cancer, particularly long-time smokers. These CT scans not only focus on identifying lung cancer nodules but also provide valuable anatomical information, including details about body composition.
The study’s lead author, Kaiwen Xu, a Ph.D. candidate at Vanderbilt University, and his colleagues have previously developed and released an AI algorithm that automatically derives body composition measurements from lung screening LDCT. Body composition, which assesses the percentage of fat, muscle, and bone in the body, is crucial for understanding various health conditions like obesity, metabolic disorders, cardiovascular disease, and chronic obstructive pulmonary disease. Additionally, it has been found to impact survival and quality of life in lung cancer patients.
In their new research, the team evaluated the additional value of AI-derived body composition measurements. They analyzed CT scans from over 20,000 individuals participating in the National Lung Screening Trial. The results showed that incorporating these measurements significantly improved risk prediction for death from lung cancer, cardiovascular disease, and all-cause mortality.
Xu emphasizes that AI-based body composition analysis extends the benefits of lung screening beyond early lung cancer detection. It enables the identification of high-risk individuals for interventions like physical conditioning or lifestyle modifications even before the onset of the disease.
Certain measurements related to fat within muscle proved to be particularly strong predictors of mortality, consistent with existing research. Myosteatosis, the infiltration of skeletal muscle with fat, is now considered a more indicative factor for health outcomes than reduced muscle bulk.
The practice of opportunistic screening, where imaging for one purpose provides information about other conditions, holds great potential for routine clinical use. CT scans ordered for lung cancer detection can also yield valuable data about body composition and coronary artery calcification associated with cardiovascular disease risk.
This study focused on individuals at a baseline screening only. For future research, the team aims to conduct a longitudinal study, following individuals over time to observe how changes in body composition relate to health outcomes.