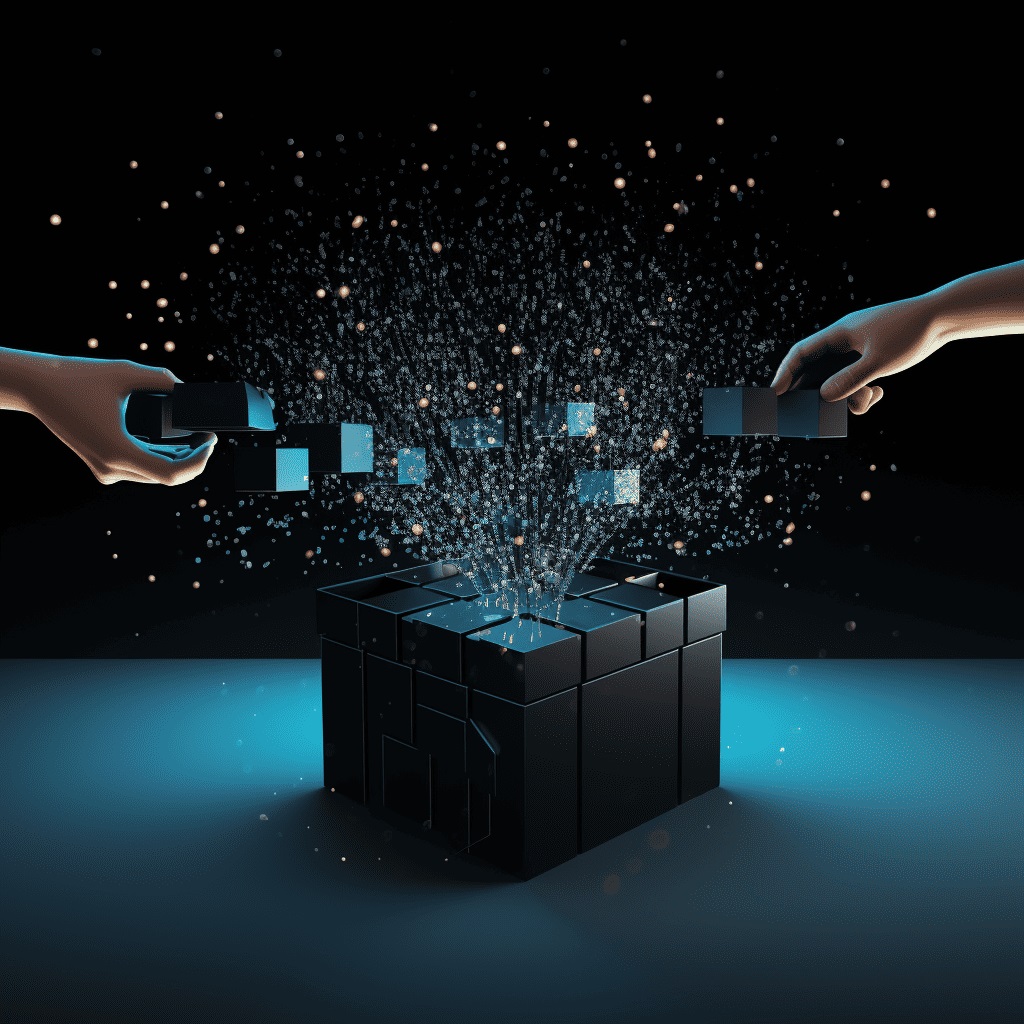
In healthcare, ‘black box’ AI models pose a challenge due to their opaque decision-making processes. A novel auditing framework, devised by Stanford University and University of Washington researchers, aims to tackle this issue. By combining human expertise with generative AI, the framework scrutinizes classifiers, revealing insights into their decision rationale. Testing on dermatology AI classifiers demonstrates the effectiveness of this approach, highlighting the interplay between desirable and undesirable features. The findings offer crucial insights for developers, aiding in the identification and resolution of spurious correlations. Ultimately, transparent AI holds the key to enhancing trust, accuracy, and patient outcomes in healthcare.
In healthcare AI, the opacity of ‘black box’ models poses a formidable challenge, hindering trust and acceptance among users. Addressing this issue, researchers from Stanford University and the University of Washington have unveiled a pioneering auditing framework. Designed to unravel the enigmatic decision-making processes of AI classifiers, the framework merges human expertise with generative AI techniques. By shedding light on the inner workings of these models, the framework aims to foster trust and enable informed decision-making. This introduction sets the stage for a discussion on the significance of transparency and accountability in medical AI.
In the realm of healthcare artificial intelligence (AI), the enigmatic nature of ‘black box’ models has long been a challenge, hindering trust and acceptance among users. However, a groundbreaking auditing framework, developed by researchers from Stanford University and the University of Washington, offers hope for unraveling the opaque decision-making processes of these AI tools.
Understanding the Black Box Problem:
The term ‘black box’ refers to the inherent opacity of AI models, where users are unable to discern how decisions are reached. This lack of transparency breeds distrust, particularly in critical domains like healthcare, where the stakes are high. The inability to scrutinize the inner workings of AI systems poses a significant hurdle to their widespread adoption.
The Quest for Explainability:
Recognizing the imperative for explainability in AI, the research team embarked on a mission to devise an auditing mechanism capable of elucidating the inference processes of healthcare AI models. This endeavor seeks to empower users with insights into how these models arrive at their conclusions, thereby fostering trust and facilitating informed decision-making.
The Auditing Framework:
At the core of the auditing framework lies a fusion of human expertise and generative AI. By leveraging this innovative approach, classifiers—algorithms tasked with categorizing data inputs—are subjected to rigorous evaluation aimed at uncovering the rationale behind their decisions. The effectiveness of this framework was put to the test through a comprehensive study involving dermatology AI classifiers.
Unveiling the Decision-Making Process:
In the dermatology domain, where AI holds promise for aiding in the diagnosis of skin conditions, the auditing framework proved instrumental in unraveling the intricacies of model decision-making. Through meticulous analysis of lesion images and collaboration with domain experts, the researchers unearthed key insights into the features influencing classifier outcomes.
Insights and Implications:
The findings shed light on the interplay between desirable and undesirable features in the classifiers’ decision-making. By identifying spurious correlations within datasets, developers can preemptively address issues that may compromise the efficacy of AI solutions in healthcare settings. Moreover, the insights garnered from the auditing process hold profound implications for the burgeoning landscape of dermatology AI.
Navigating the Challenges:
As healthcare AI continues to proliferate, concerns surrounding the ‘black box’ problem loom large. The emergence of direct-to-consumer apps further underscores the urgency of enhancing transparency and accountability in AI algorithms. With a clearer understanding of AI decision-making, consumers can make informed choices and developers can refine their models to prioritize clinically relevant features.
Fostering Confidence and Accuracy:
Explainable AI approaches stand as linchpins in fortifying the accuracy of medical AI classifiers and instilling confidence among users. By demystifying the decision-making processes, these approaches pave the way for transformative advancements in healthcare. Dr. Roxana Daneshjou, a senior study co-author, emphasizes the pivotal role of transparent AI in driving improvements in patient outcomes.
Addressing Clinician Concerns:
The advent of AI in healthcare inevitably raises questions regarding clinician reliance on these tools. Experts emphasize the importance of mitigating over-reliance through education and vigilance. By fostering a culture of critical thinking and augmenting AI with human expertise, healthcare organizations can harness the full potential of these technologies while safeguarding against potential pitfalls.
The unveiling of an auditing framework marks a significant milestone in the quest to demystify medical AI’s ‘black box.’ By bridging the gap between users and AI systems, this framework heralds a new era of transparency and accountability in healthcare. With stakeholders united in their commitment to elucidating AI’s inner workings, the promise of transformative innovation in medicine shines ever brighter. As developers refine their models and users gain insights into AI decision-making, the stage is set for enhanced trust, accuracy, and ultimately, improved patient outcomes. Transparent AI stands as a beacon of progress in the evolving landscape of healthcare technology.