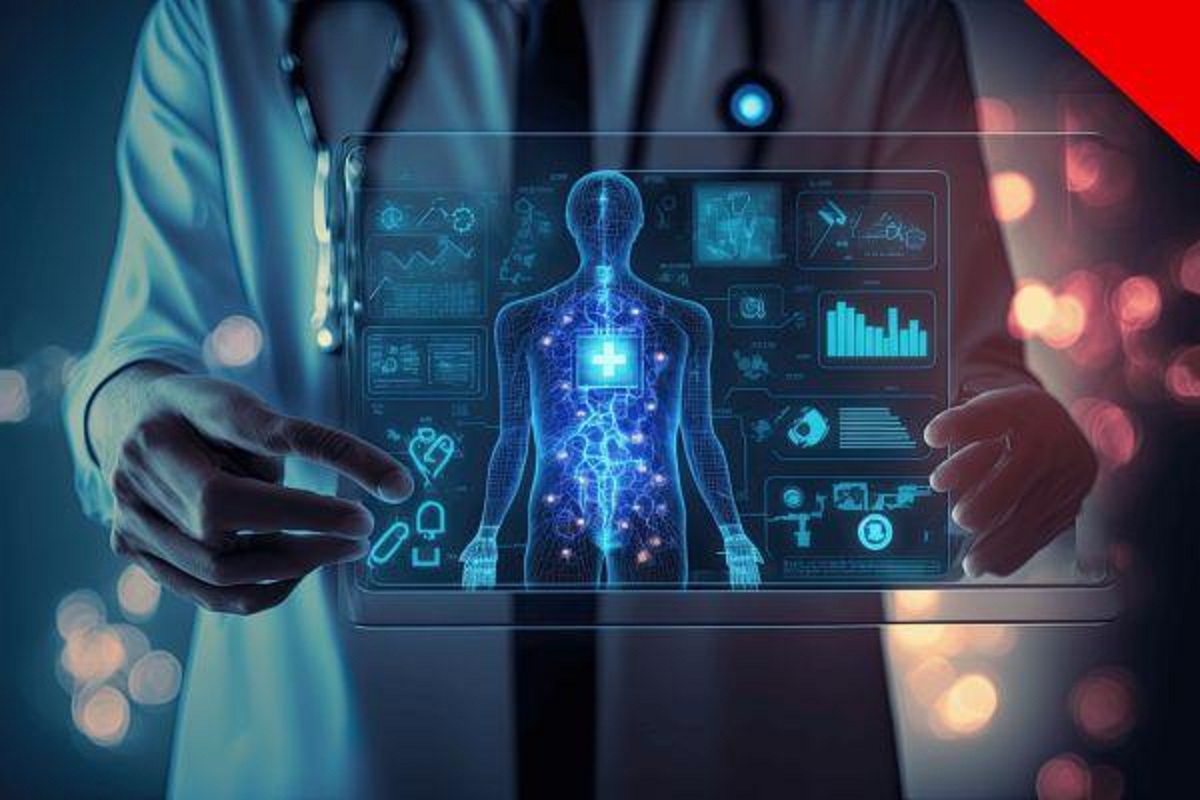
In the realm of healthcare, the integration of artificial intelligence (AI) and machine learning represents a pivotal advancement in early detection and management of clinical deterioration. Recent studies underscore the transformative potential of these technologies in mitigating risks associated with patient care escalations during hospitalization. By harnessing sophisticated algorithms and predictive analytics, AI-driven interventions offer healthcare professionals invaluable insights into identifying and addressing early warning signs of deterioration. This not only leads to a significant reduction in adverse events but also enhances patient safety and outcomes. The ongoing exploration and implementation of AI and machine learning in healthcare practice are essential for realizing their full potential in revolutionizing patient care delivery.
In the dynamic landscape of healthcare, the utilization of artificial intelligence (AI) and machine learning has emerged as a game-changer in the proactive management of clinical deterioration. The early identification of subtle indicators of patient decline poses a considerable challenge for healthcare providers, often resulting in adverse outcomes. However, recent advancements in AI technology have paved the way for innovative interventions designed to detect and address these warning signs promptly. By leveraging vast datasets and sophisticated algorithms, AI-enabled tools offer unprecedented capabilities in predicting and mitigating care escalations during hospitalization. This introduction explores the transformative potential of AI and machine learning in revolutionizing patient care by enhancing early detection and intervention strategies, ultimately leading to improved outcomes and enhanced patient safety.
Addressing Clinical Deterioration with Artificial Intelligence
Clinical deterioration poses a significant challenge in healthcare, often leading to adverse outcomes for patients. However, identifying early warning signs of deterioration remains complex, contributing to a notable percentage of avoidable deaths in hospitals. In response to this pressing issue, researchers have explored innovative approaches leveraging artificial intelligence (AI) to detect and mitigate clinical deterioration effectively.
Study Overview
A recent study, published in JAMA Internal Medicine, sheds light on the efficacy of an AI-driven clinical deterioration intervention in reducing the risk of care escalations during hospitalization. The research aimed to evaluate the impact of an AI model designed to detect clinical deterioration on inpatient care escalation rates, thereby addressing a critical gap in current healthcare practices.
Assessing AI Intervention
The study analyzed data from 9,938 hospitalized patients across four internal medicine units within an academic medical center. The intervention utilized an AI-enabled tool, the Epic Deterioration Index (EDI), which generated alerts based on clinical indicators of deterioration. These alerts triggered a predefined clinical workflow aimed at mitigating escalation events.
Reduction in Care Escalations
Results indicated a notable reduction in the risk of care escalations associated with the AI intervention. Specifically, there was an absolute risk reduction of 10.4 percentage points in care escalations among patients identified at the EDI score threshold. This finding underscores the potential of AI-driven interventions in significantly mitigating the risks associated with clinical deterioration during hospitalization.
Implications and Future Directions
The study’s findings hold significant implications for healthcare practice, highlighting the effectiveness of AI-driven interventions in enhancing patient care and safety. Moreover, the success of this intervention underscores the need for further exploration and implementation of similar models across various care settings. Future research endeavors should focus on assessing the scalability and generalizability of AI-driven interventions in diverse healthcare contexts.
Leveraging Machine Learning to Predict Pediatric Deterioration
In addition to adult populations, pediatric patients also face risks associated with clinical deterioration during hospitalization. Recognizing the importance of early detection in pediatric care, researchers have developed machine learning models tailored to predict the risk of deterioration among pediatric patients.
Study Insights
A recent study conducted at Nationwide Children’s Hospital showcased the effectiveness of a machine learning model in predicting the risk of pediatric deterioration. The model, utilizing the Deterioration Risk Index (DRI), demonstrated superior accuracy and precision compared to existing tools.
Enhanced Accuracy and Precision
The machine learning model exhibited 2.4 times greater sensitivity overall, with particularly significant improvements in sensitivity for cardiac and malignancy-related deterioration. Furthermore, the model required fewer alerts per detected event, indicating enhanced efficiency in identifying at-risk patients.
Clinical Implementation and Impact
Following a successful pilot project deploying the machine learning model in a clinical setting, researchers observed a substantial reduction (77%) in deterioration events over 18 months. This significant reduction underscores the potential of machine learning-driven interventions in improving patient outcomes and safety in pediatric healthcare settings.
The integration of artificial intelligence (AI) and machine learning stands as a beacon of hope in the landscape of healthcare, offering unprecedented opportunities to enhance patient care and safety. The evidence presented in recent studies underscores the profound impact of these technologies in reducing care escalations and adverse events during hospitalization. By leveraging advanced algorithms and predictive analytics, AI-driven interventions empower healthcare professionals to detect and address clinical deterioration with unparalleled precision and timeliness. However, while the results are promising, further research and implementation efforts are imperative to optimize the effectiveness and scalability of AI and machine learning solutions in diverse healthcare settings. With continued dedication and innovation, these technologies hold the potential to revolutionize healthcare delivery, ultimately improving patient outcomes and transforming the future of medicine.