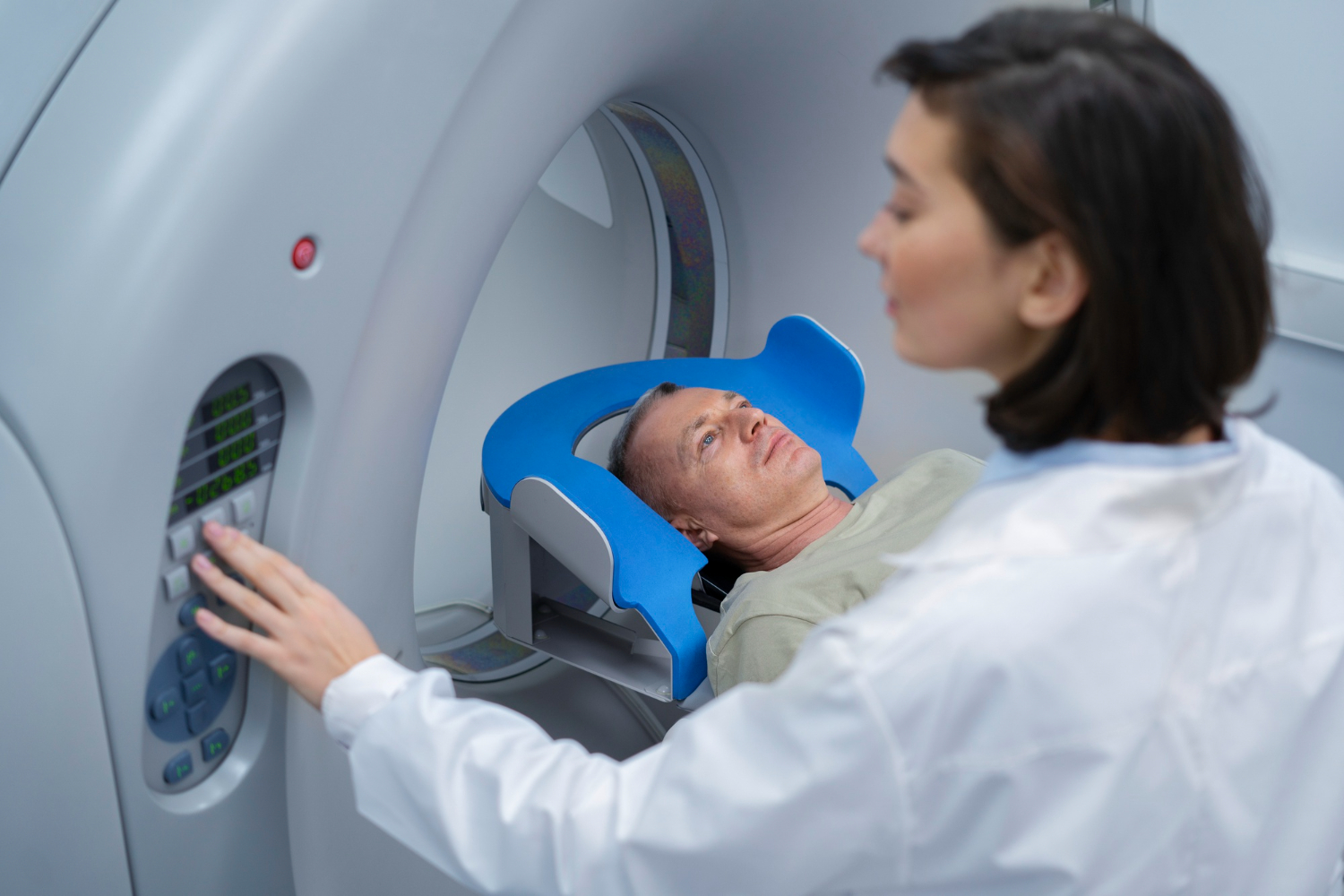
Breast cancer remains one of the leading causes of death among women worldwide. The detection and treatment of metastasis, particularly in the axillary lymph nodes, is crucial for improving patient outcomes. Recent advancements in MRI-based machine learning approaches have shown promising results in predicting breast cancer metastasis, thereby reducing the need for invasive biopsies. This blog explores the groundbreaking research from UT Southwestern Medical Center that uses a convolutional neural network to flag axillary metastasis with remarkable accuracy.
Overview of MRI-based Machine Learning in Breast Cancer Detection
MRI-based machine learning approaches have revolutionized the medical imaging field, offering new ways to diagnose and predict disease progression. Specifically, in breast cancer detection, these approaches leverage the power of advanced algorithms to analyze complex imaging data, providing insights that go beyond traditional diagnostic methods.
The Role of Convolutional Neural Networks
A convolutional neural network (CNN) is a type of deep learning algorithm particularly effective in processing and analyzing visual data. In the context of breast cancer detection, CNNs are used to scrutinize MRI images, identifying patterns and anomalies that may indicate the presence of cancer cells in the axillary lymph nodes. This method offers a non-invasive alternative to traditional biopsies, significantly reducing the physical and emotional burden on patients.
Methodology and Research Findings
Data Collection
The study conducted by UT Southwestern Medical Center utilized data from 350 newly diagnosed breast cancer patients with known nodal status. The MRI images and clinical data were fed into a four-dimensional convolutional neural network designed to detect axillary metastasis.
Model Performance
The results of the study were impressive. The model achieved an area under the receiver operating characteristic curve (AUC) of 0.87, with a sensitivity of 89% and a specificity of 76%. Furthermore, the tool accurately identified 95% of patients with axillary metastasis, demonstrating its potential to reduce unnecessary surgical biopsies.
The model’s performance was consistent across different datasets. When tested on data from a safety-net hospital, it achieved an AUC of 0.84. The performance slightly dipped to an AUC of 0.81 when tested on data from a university hospital, but the overall accuracy remained high.
Clinical Implications and Benefits
The ability to accurately predict axillary metastasis using MRI-based machine learning has significant clinical implications. Traditional imaging techniques often lack the sensitivity required to rule out metastasis, leading to invasive procedures such as sentinel lymph node biopsies. These procedures, while necessary, carry risks and side effects. The new tool developed by UT Southwestern Medical Center can help avoid these procedures, improving patient outcomes and reducing healthcare costs.
Comparison with Traditional Methods
Traditional methods for detecting breast cancer metastasis often involve a combination of imaging techniques and surgical biopsies. While effective, these methods can be invasive, time-consuming, and stressful for patients. In contrast, the MRI-based machine learning approach offers a non-invasive, efficient, and highly accurate alternative. By integrating clinical data with advanced imaging techniques, this approach enhances the detection process, providing more reliable results.
Future Directions in AI and Breast Cancer Research
The success of the MRI-based machine learning approach in predicting breast cancer metastasis opens new avenues for research and development. Future studies could focus on refining the algorithm, expanding its application to other types of cancer, and integrating it with other diagnostic tools. Additionally, further research is needed to explore the long-term benefits and potential challenges of implementing such technology in clinical practice.
Conclusion
The development of an MRI-based machine learning approach to predict breast cancer metastasis represents a significant advancement in cancer detection and treatment. By accurately identifying axillary metastasis, this tool has the potential to reduce unnecessary biopsies, improve patient outcomes, and streamline the diagnostic process. As research continues to evolve, the integration of AI in medical imaging promises to transform the landscape of cancer care.
Discover the latest Provider news updates with a single click. Follow DistilINFO HospitalIT and stay ahead with updates. Join our community today!
Frequently Asked Questions (FAQs)
What is an MRI-based machine learning approach?
An MRI-based machine learning approach uses algorithms to analyze MRI images and predict disease progression, offering a non-invasive diagnostic alternative.
How does the convolutional neural network work in detecting breast cancer?
The convolutional neural network processes and analyzes MRI images, identifying patterns that indicate the presence of cancer cells in the axillary lymph nodes.
What are the benefits of using this tool over traditional methods?
This tool reduces the need for invasive biopsies, offers higher accuracy in detecting metastasis, and improves patient outcomes by providing a non-invasive diagnostic option.
By leveraging advanced MRI-based machine learning techniques, we are stepping into a new era of breast cancer detection and treatment, promising a brighter future for patients and healthcare providers alike.