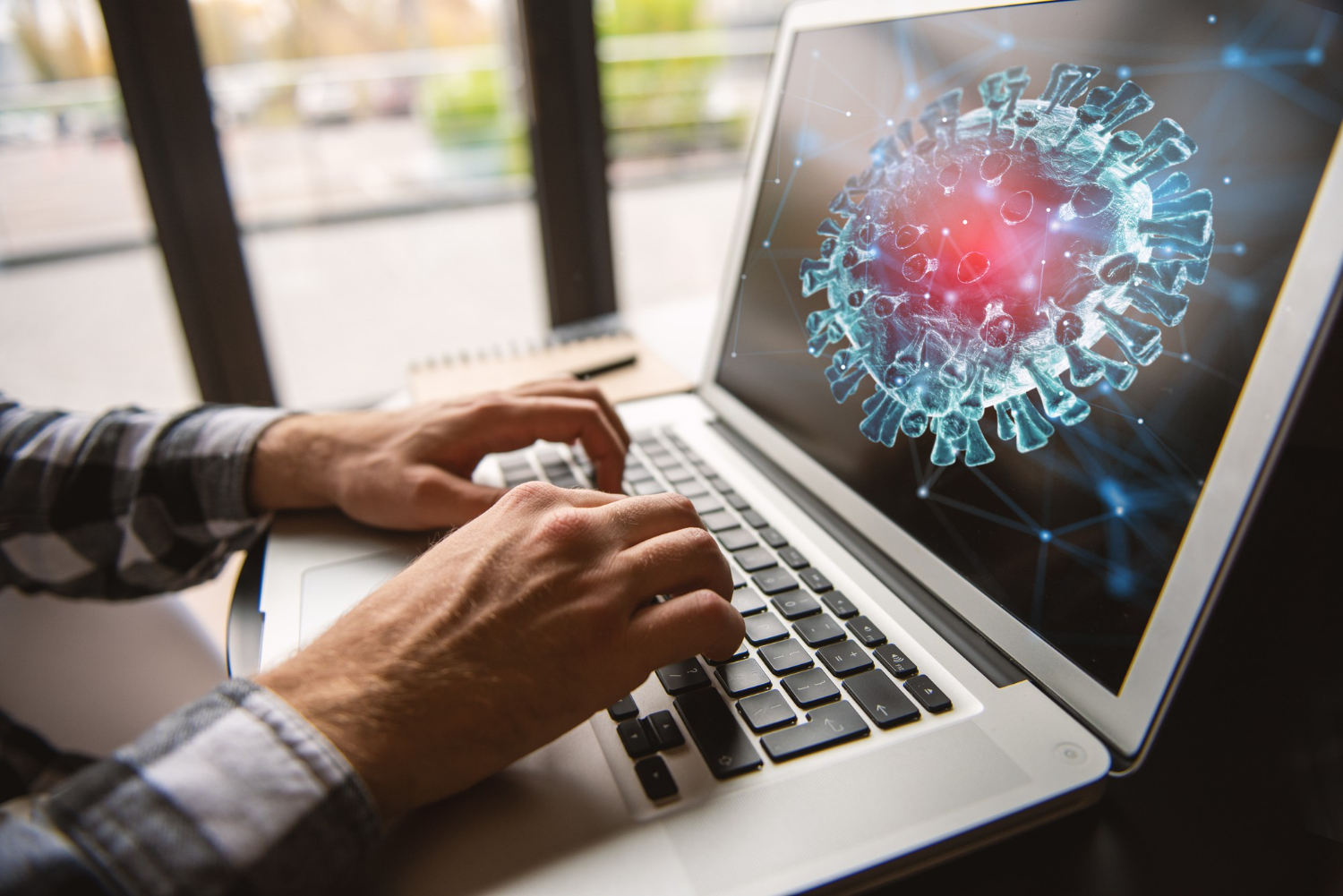
Introduction
In the realm of cancer detection, artificial intelligence (AI) has shown immense promise. However, the development of effective AI algorithms depends heavily on the availability of high-quality, annotated medical data. A groundbreaking method developed by a research team led by Johns Hopkins University aims to address this challenge by generating large datasets of synthetic liver tumor computed tomography (CT) scans. This innovative approach could significantly enhance the training of AI models for cancer detection.
The Need for Synthetic Tumor Data
Flagging tumors on medical scans is a complex and time-consuming process, often requiring detailed interpretation of pathology reports and biopsy confirmations. This meticulous process makes it difficult to curate large-scale datasets necessary for AI training. Currently, there are only about 200 publicly available annotated CT scans of liver tumors, a number insufficient for robust AI model training and testing.
Four-Step Framework for Generating Synthetic Tumors
To address the scarcity of high-quality annotated data, the research team developed a four-step framework to generate synthetic tumors that are nearly indistinguishable from real ones.
Choosing Tumor Locations
The first step involves selecting locations for artificial tumors that avoid collisions with surrounding blood vessels. This careful placement is crucial to ensure that the synthetic tumors appear realistic and anatomically plausible.
Adding Random Noise
Next, the team added random “noise” to the data to simulate the irregular textures characteristic of real tumors. This step enhances the realism of the synthetic images by mimicking the natural variations found in actual tumor tissues.
Generating Realistic Shapes
The third step focuses on generating shapes that mimic the diverse forms of real tumors. Tumors can vary greatly in shape and size, and capturing this diversity is essential for training AI to recognize a wide range of tumor presentations.
Simulating Tumor Appearance Changes
Lastly, the researchers simulated changes in tumor appearance resulting from their tendency to push on and displace surrounding tissues. This step ensures that the synthetic tumors exhibit the same dynamic interactions with their environment as real tumors do.
Training AI with Synthetic Tumor Data
The synthetic tumors generated using this method were put to the test by training an AI algorithm exclusively on this data. Remarkably, the AI model trained with synthetic tumor data outperformed similar approaches and achieved results comparable to models trained on real tumor data. Qixin Hu, a researcher from the Huazhong University of Science and Technology, highlighted the significance of this achievement, noting that it is the first time synthetic tumors alone have yielded such high-performance AI results.
Expanding to Other Types of Cancer
The research team is not stopping at liver tumors. They are currently exploring advanced image processing techniques to generate synthetic tumors for other organs, including the pancreas and kidneys. The ultimate goal is to create comprehensive datasets encompassing all types of tumors and abnormalities, thereby facilitating the development of AI models across various medical conditions.
Benefits and Challenges of Synthetic Healthcare Data
The use of synthetic healthcare data presents several benefits, including enhanced privacy and the ability to generate large-scale datasets without relying on real patient information. However, challenges remain, particularly regarding the quality and realism of synthetic data. Ongoing research and technological advancements aim to address these issues, improving the utility and reliability of synthetic data in medical research and AI development.
Conclusion
The method developed by the Johns Hopkins University-led team marks a significant step forward in the field of cancer detection AI. By generating high-quality synthetic tumor data, researchers can overcome the limitations posed by the scarcity of annotated medical images. This innovative approach holds the potential to revolutionize AI training for cancer detection and pave the way for advancements in other areas of medical imaging and diagnosis.
Discover the latest Provider news updates with a single click. Follow DistilINFO HospitalIT and stay ahead with updates on medical advancements. Join our community today!
FAQs
What is synthetic tumor data?
Synthetic tumor data refers to artificially generated medical images of tumors, designed to mimic the appearance and characteristics of real tumors. This data is used to train AI algorithms for cancer detection.
Why is synthetic tumor data important?
Synthetic tumor data is crucial for training AI models, especially when there is a scarcity of high-quality, annotated real medical images. It allows researchers to create large datasets needed for effective AI training.
How does the synthetic tumor generation process work?
The process involves a four-step framework: choosing tumor locations to avoid anatomical collisions, adding random noise to simulate texture, generating realistic shapes, and simulating changes in appearance due to tissue displacement.
Can synthetic data be used for other types of cancer?
Yes, the research team is exploring the generation of synthetic tumors for other organs, such as the pancreas and kidneys, with the goal of creating comprehensive datasets for various types of cancer.
What are the benefits of using synthetic healthcare data?
Synthetic healthcare data enhances privacy by eliminating the need for real patient information and enables the creation of large, high-quality datasets for AI training, improving the accuracy and performance of AI models.
Leave a Reply