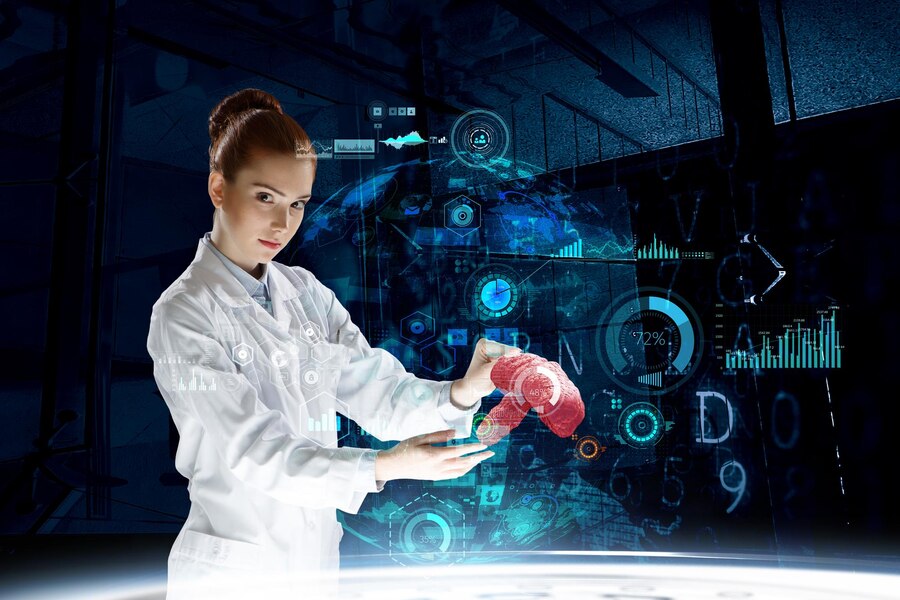
Medical Testing in AI : Introduction
Medical Testing in AI plays a vital role in promoting bias-free healthcare systems by addressing data gaps that could lead to disparities. Inequities in Medical Testing, particularly across racial and socioeconomic groups, contribute to biased data, which can influence its clinical predictions. A recent study published in PLOS Global Public Health highlights how differences in Medical Testing rates for Black and white patients can lead to biased data sets, which in turn create racial biases within AI models. By focusing on equitable medical testing and using robust data correction methods, healthcare providers and AI developers can create more accurate, inclusive models.
Understanding Racial Bias in Medical Testing
Healthcare disparities based on race have been well-documented, but the role of Medical Testing inequities in creating biased Artificial intelligence models is a newer concern. Black patients are often less likely to receive diagnostic testing for severe illnesses such as sepsis compared to white patients. This discrepancy means that data from Black patients is underrepresented in health records, leading to a gap in AI training data.
Impact of Medical Testing Inequities on AI Training Data
Inequities in Medical Testing directly influence the quality of it training data. When diagnostic tests are not conducted uniformly across racial groups, the data used to train the models reflects these disparities. This can result in Artificial intelligence models that underperform for Black patients and overestimate outcomes for others, leading to incorrect or biased clinical predictions.
Artificial intelligence Bias in Clinical Decision Support Systems
AI in clinical decision support systems (CDSS) assists clinicians in diagnosing and managing diseases by providing predictions based on patient data. However, if it models are trained on biased data, they can reinforce existing health disparities. For instance, when an AI tool is trained on data that underrepresents Black patients due to lower testing rates, the model may be less accurate in identifying illnesses in Black populations. This lack of accuracy makes it difficult for CDSS to support equitable healthcare.
Research Findings on Testing Inequities and AI Bias
The recent research in PLOS Global Public Health used data from hospitals to examine how testing inequities contribute to bias in Artificial Intelligence. When diagnostic test rates for Black and white patients were compared, the differences showed a clear pattern of under-testing among Black patients. This disparity then impacts Artificial intelligence models trained on such data, potentially leading to biased predictions that underestimate the severity of illness in Black populations.
Algorithmic Approaches to Reducing Artificial intelligence Bias
To counteract the effects of testing inequities, the research team developed a computer algorithm that could help AI models account for patients who may have been under-tested. By simulating a data set where certain patients were reclassified based on predicted likelihoods of illness, the team demonstrated that algorithmic adjustments could improve Artificial intelligence model accuracy. When bias correction techniques were applied, the AI was able to more accurately predict severe illness cases in Black patients, improving equity in clinical decision support.
Challenges in Addressing AI Bias in Healthcare
While adjusting the models for bias is essential, it presents several challenges. Omitting patient records to create a “clean” data set can lead to models that lack accuracy for less severely ill patients. Additionally, removing data points based on race or socioeconomic status can dilute the nuances that are critical for effective healthcare. Therefore, it is essential to find solutions that correct for bias without compromising the model’s robustness or accuracy.
Potential Solutions to Correct Data Bias in AI Models
Several methods can address data bias in AI without compromising the accuracy or inclusiveness of the model:
Limiting Data Bias without Omitting Records
One approach is to adjust data sets by simulating outcomes for patients who may have been under-tested. By reclassifying certain patients based on other health indicators like vital signs, the developers can approximate a more balanced data set without omitting records. This approach can help build models that reflect a realistic and fair distribution of health conditions across racial groups.
Importance of Adjusting for Systematic Bias
AI models can also incorporate statistical techniques that adjust for systematic bias. This includes controlling for factors such as admission rates and historical under-testing patterns. By acknowledging and adjusting for these factors, models can better represent diverse patient populations, helping ensure more equitable health predictions.
Conclusion
The study in PLOS Global Public Health underscores the critical need to address racial bias in AI-enabled healthcare. Inequities in diagnostic testing contribute to biased data sets, which can reinforce racial disparities in healthcare outcomes. As healthcare systems increasingly turn to Artificial intelligence, it is essential to develop models that accurately reflect diverse populations and provide fair, equitable predictions. Addressing data bias is a necessary step toward using it in ways that promote health equity, ultimately leading to better and more inclusive healthcare.
Discover the latest Provider news updates with a single click. Follow DistilINFO HospitalIT and stay ahead with updates on medical advancements. Join our community today!
FAQs
1. How does Medical Testing inequity contribute to Artificial intelligence bias?
Ans: When certain racial groups receive fewer Medical Testing, the data used to train it reflects this disparity, leading to biased predictions that may underrepresent illness in those populations.
2. What did the recent study find about Medical Testing rates?
Ans: The study found that Black patients were less likely to receive diagnostic tests than white patients, which contributes to biased Artificial intelligence models in healthcare.
3.How can Artificial intelligence bias be corrected without removing records?
Ans: Algorithms can be adjusted to account for under-tested groups, simulating balanced data sets without omitting any records, thereby improving model accuracy.