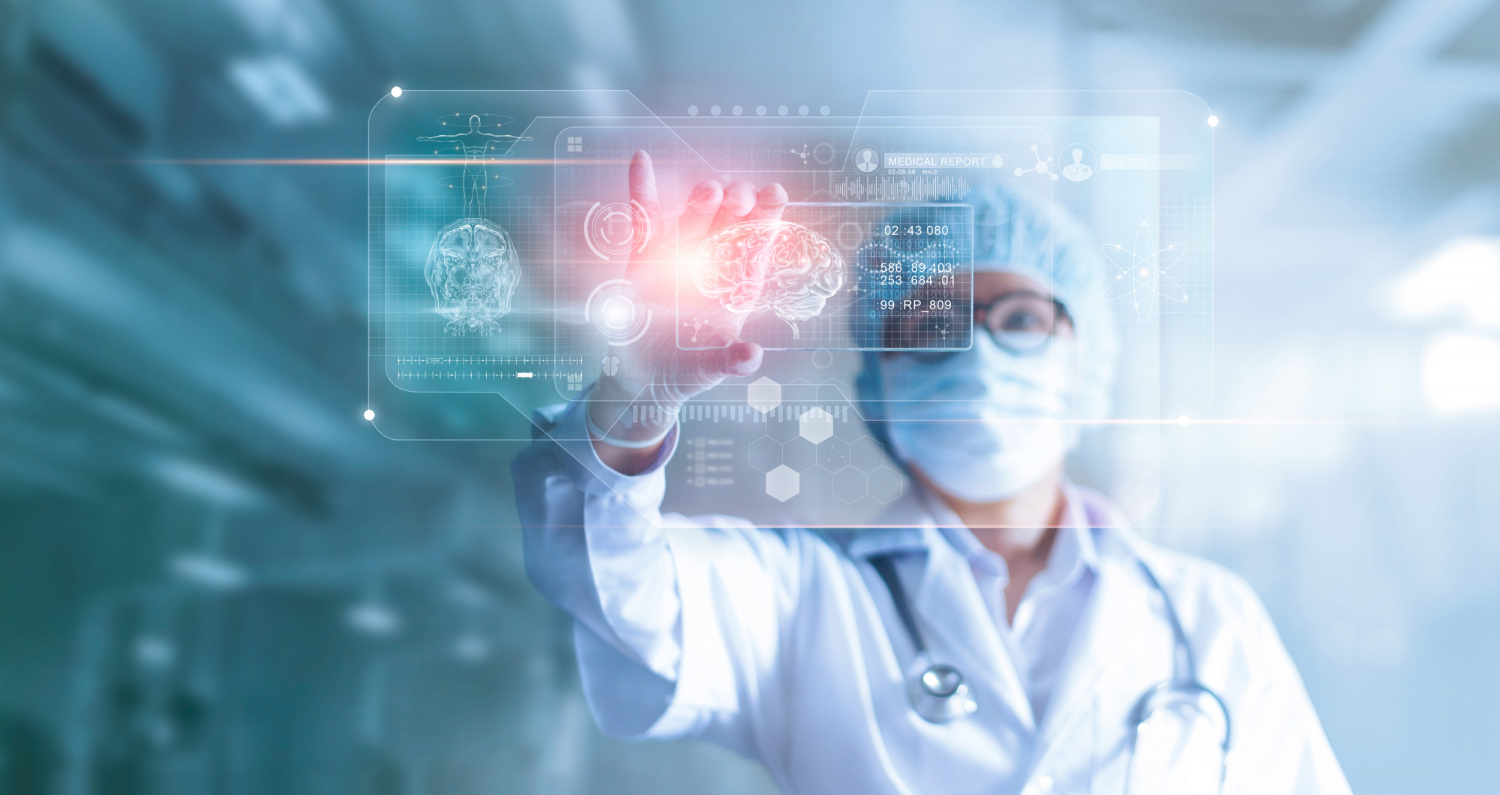
Understanding AI Governance in Healthcare
Healthcare organizations must carefully evaluate artificial intelligence technologies within a comprehensive governance framework to ensure optimal patient outcomes. As AI systems become increasingly integrated into healthcare operations, establishing robust evaluation processes for vendor-provided tools has become crucial for maintaining safety, effectiveness, and ethical standards.
Key Risk Categories in AI Evaluation
Healthcare systems must implement effective mechanisms for assessing and understanding risks associated with clinical AI implementations. The evaluation framework encompasses four critical risk categories:
Correctness and Transparency Assessment
Organizations must verify the accuracy of AI algorithms and ensure transparency in their decision-making processes. This involves thorough testing and validation of results across different scenarios and patient populations.
Fairness and Equity Considerations
AI systems must demonstrate unbiased performance across diverse patient demographics. Healthcare providers need to evaluate potential disparities in system outcomes and ensure equitable care delivery.
Workflow Integration Analysis
Successful AI implementation requires seamless integration with existing clinical workflows. Organizations should assess how AI tools complement and enhance current processes without disrupting patient care.
Safety and Privacy Protocols
Protecting patient data and ensuring system safety are paramount. Healthcare providers must evaluate vendor compliance with privacy regulations and implement robust security measures.
Expert Insights on AI Governance
Glenn Wasson, Analytics Administrator at UVA Health and computer science Ph.D., emphasizes the importance of establishing strong governance frameworks for commercial AI systems. His upcoming HIMSS25 session, “Dear AI Vendors: This Is What We Need,” will address critical aspects of AI vendor evaluation.
Novel Risks and Evaluation Challenges
Modern AI systems present unique challenges compared to traditional software implementations. Healthcare organizations must develop new evaluation frameworks that address:
- Complex risk assessment requirements
- Limited access to vendor code
- Need for increased transparency
- Ongoing monitoring of AI system performance
Building Provider-Vendor Relationships
Effective AI governance requires open dialogue between healthcare providers and vendors. This communication should focus on:
- Understanding data sources and algorithms
- Evaluating workflow integration
- Assessing risk mitigation strategies
- Maintaining continuous system monitoring
Implementation Considerations
Healthcare organizations must involve various stakeholders in AI evaluation, including:
- Clinical leaders
- Operational staff
- Technical experts
- Workflow specialists
The dynamic nature of AI systems requires ongoing evaluation and adjustment of governance frameworks to ensure continued effectiveness and safety.
Discover the latest Provider news updates with a single click. Follow DistilINFO HospitalIT and stay ahead with updates. Join our community today!
Leave a Reply